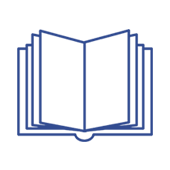
Hybrid Methods of GMDH-Neural Networks Synthesis and Training for Solving Problems of Time Series Forecasting
Fragment książki (Rozdział monografii pokonferencyjnej)
MNiSW
20
Poziom I
Status: | |
Autorzy: | Lytvynenko Volodymyr I., Wójcik Waldemar, Fefelov Andrey , Lurie Iryna, Savina Nataliia B., Voronenko Mariia, Boskin Oleg, Smailova Saule |
Dyscypliny: | |
Aby zobaczyć szczegóły należy się zalogować. | |
Wersja dokumentu: | Drukowana | Elektroniczna |
Arkusze wydawnicze: | 1,2 |
Język: | angielski |
Strony: | 513 - 531 |
Web of Science® Times Cited: | 10 |
Scopus® Cytowania: | 30 |
Bazy: | Web of Science | Scopus |
Efekt badań statutowych | TAK |
Materiał konferencyjny: | NIE |
Publikacja OA: | NIE |
Abstrakty: | angielski |
In this paper, for solving the problem of forecasting non-stationary time series, hybrid learning methods for GMDH-neural networks are proposed. Training methods combine artificial immune systems with members of the evolutionary algorithm family, in particular, gene expression programming systems. The following hybrid computational methods for the synthesis and training of GMDH-neural networks have been developed: a method in which candidate models are represented as gene expression, and training is performed by clonal selection; the method of two-phase structural-parametric synthesis, in which the structural component is formed by programming the expression of genes, and the parameterization is performed by clonal selection; a method based on cooperative-competitive processes of interaction of the elements of the immune system, in which the structure and parameters of the GMDH-neural network are represented by the entire population element-wise. Comparative experimental studies of the quality of the proposed computational methods for solving forecasting problems were carried out. |