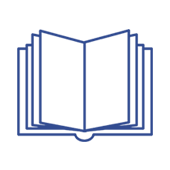
Multi-class classification of EEG spectral data for artifact detection
Fragment książki (Rozdział monografii pokonferencyjnej)
MNiSW
20
Poziom I
Status: | |
Autorzy: | Tokovarov Mikhail, Plechawska-Wójcik Małgorzata, Kaczorowska Monika |
Dyscypliny: | |
Wersja dokumentu: | Drukowana | Elektroniczna |
Język: | angielski |
Strony: | 305 - 316 |
Web of Science® Times Cited: | 1 |
Scopus® Cytowania: | 1 |
Bazy: | Web of Science | Scopus |
Efekt badań statutowych | NIE |
Materiał konferencyjny: | NIE |
Publikacja OA: | NIE |
Abstrakty: | angielski |