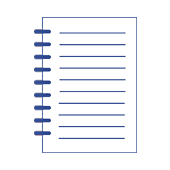
Surface quality simulation with neural networks in AZ91D Mg alloy milling
Materiały konferencyjne
MNiSW
5
spoza listy
Status: | |
Autorzy: | Kulisz Monika, Zagórski Ireneusz, Korpysa Jarosław |
Dyscypliny: | |
Aby zobaczyć szczegóły należy się zalogować. | |
Rok wydania: | 2019 |
Wersja dokumentu: | Elektroniczna |
Język: | angielski |
Numer czasopisma: | 1 |
Wolumen/Tom: | 710 |
Numer artykułu: | 012019 |
Strony: | 1 - 11 |
Web of Science® Times Cited: | 1 |
Scopus® Cytowania: | 1 |
Bazy: | Web of Science | Scopus |
Efekt badań statutowych | NIE |
Materiał konferencyjny: | TAK |
Nazwa konferencji: | IV International Conference of Computational Methods in Engineering Science - CMES'19 |
Skrócona nazwa konferencji: | CMES'19 |
Termin konferencji: | 21 listopada 2019 do 23 listopada 2019 |
Miasto konferencji: | Kazimierz Dolny |
Państwo konferencji: | POLSKA |
Publikacja OA: | TAK |
Licencja: | |
Sposób udostępnienia: | Witryna wydawcy |
Wersja tekstu: | Ostateczna wersja opublikowana |
Czas opublikowania: | W momencie opublikowania |
Data opublikowania w OA: | 19 grudnia 2019 |
Abstrakty: | angielski |
The mathematical model presented in this paper describes the process of milling AZ91D Mg alloy with the TiAlN-coated tool. The experimental data from the neural network training served to determine the effect of various milling parameters on the surface roughness of the workpiece. The 2D roughness measurements were taken on the end-faces of specimens and focused on the set of three parameters – Ra, Rz and RSm. The tests were performed at constant parameters (tool geometry, workpiece strength properties and technological machine properties), variable parameters (cutting speed, feed per tooth, depth of cut) and output variables (Ra, Rz, Rsm). The simulations were executed by means of the artificial neural networks, modelled with Statistica Neural Network software. Two types of neural networks were employed: MLP (Multilayered Perceptron) and RBF (Radial Basis Function). The comparative analysis showed that the discrepancy between the results of simulation and the results obtained from experimental tests is on an acceptable level, not exceeding 15%. The above result confirms that ANNs can be successfully used as a tool for a selection of technological parameters, enabling obtaining the desired values of surface roughness parameters without the need for time-consuming machining tests. |