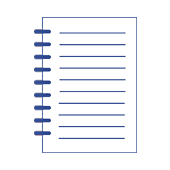
Single channel source separation with ICA-based time-frequency decomposition
Artykuł w czasopiśmie
MNiSW
100
Lista 2021
Status: | |
Autorzy: | Mika Dariusz, Budzik Grzegorz, Józwik Jerzy |
Dyscypliny: | |
Aby zobaczyć szczegóły należy się zalogować. | |
Rok wydania: | 2020 |
Wersja dokumentu: | Drukowana | Elektroniczna |
Język: | angielski |
Numer czasopisma: | 7 |
Wolumen/Tom: | 20 |
Numer artykułu: | 2019 |
Strony: | 1 - 16 |
Impact Factor: | 3,576 |
Web of Science® Times Cited: | 13 |
Scopus® Cytowania: | 15 |
Bazy: | Web of Science | Scopus | DOAJ |
Efekt badań statutowych | NIE |
Finansowanie: | The project/research was financed in the framework of the project Lublin University of Technology; Regional Excellence Initiative, funded by the Polish Ministry of Science and Higher Education (contract no.030/RID/2018/19). |
Materiał konferencyjny: | NIE |
Publikacja OA: | TAK |
Licencja: | |
Sposób udostępnienia: | Otwarte czasopismo |
Wersja tekstu: | Ostateczna wersja opublikowana |
Czas opublikowania: | W momencie opublikowania |
Data opublikowania w OA: | 3 kwietnia 2020 |
Abstrakty: | angielski |
This paper relates to the separation of single channel source signals from a single mixed signal by means of independent component analysis (ICA). The proposed idea lies in a time-frequency representation of the mixed signal and the use of ICA on spectral rows corresponding to different time intervals. In our approach, in order to reconstruct true sources, we proposed a novelty idea of grouping statistically independent time-frequency domain (TFD) components of the mixed signal obtained by ICA. The TFD components are grouped by hierarchical clustering and k-mean partitional clustering. The distance between TFD components is measured with the classical Euclidean distance and the β distance of Gaussian distribution introduced by as. In addition, the TFD components are grouped by minimizing the negentropy of reconstructed constituent signals. The proposed method was used to separate source signals from single audio mixes of two- and three-component signals. The separation was performed using algorithms written by the authors in Matlab. The quality of obtained separation results was evaluated by perceptual tests. The tests showed that the automated separation requires qualitative information about time-frequency characteristics of constituent signals. The best separation results were obtained with the use of the β distance of Gaussian distribution, a distance measure based on the knowledge of the statistical nature of spectra of original constituent signals of the mixed signal. |