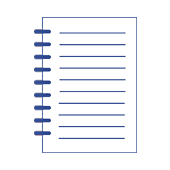
Optimum choice of signals' features used in toothed gears' diagnosis
Artykuł w czasopiśmie
Status: | |
Warianty tytułu: |
Optymalny wybór cech sygnałów wykorzystywanych w diagnozowaniu przekładni zębatych
|
Autorzy: | Jedliński Łukasz, Jonak Józef |
Rok wydania: | 2010 |
Wersja dokumentu: | Drukowana | Elektroniczna |
Język: | angielski |
Numer czasopisma: | 3 |
Wolumen/Tom: | 55 |
Strony: | 9 - 12 |
Bazy: | BazTech |
Efekt badań statutowych | NIE |
Materiał konferencyjny: | NIE |
Publikacja OA: | NIE |
Abstrakty: | angielski | polski |