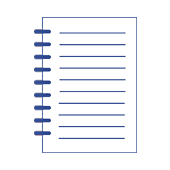
Prognozowanie wytrzymałości połączeń klejowych przy wykorzystaniu sieci neuronowych
Artykuł w czasopiśmie
Status: | |
Warianty tytułu: |
Predicting the strength of adhesive joints using neural networks
|
Autorzy: | Domińczuk Jacek |
Rok wydania: | 2010 |
Wersja dokumentu: | Drukowana | Elektroniczna |
Język: | polski |
Numer czasopisma: | 2 |
Strony: | 41 - 47 |
Bazy: | BazTech |
Efekt badań statutowych | NIE |
Materiał konferencyjny: | NIE |
Publikacja OA: | NIE |
Abstrakty: | angielski |