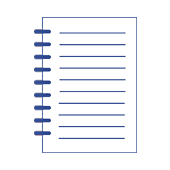
Verification of the technical equipment degradation method using a hybrid reinforcement learning trees–artificial neural network system
Artykuł w czasopiśmie
MNiSW
200
Lista 2021
Status: | |
Autorzy: | Gajewski Jakub, Vališ David |
Dyscypliny: | |
Rok wydania: | 2021 |
Wersja dokumentu: | Drukowana | Elektroniczna |
Język: | angielski |
Wolumen/Tom: | 153 |
Numer artykułu: | 106618 |
Strony: | 1 - 11 |
Impact Factor: | 5,62 |
Web of Science® Times Cited: | 20 |
Scopus® Cytowania: | 21 |
Bazy: | Web of Science | Scopus |
Efekt badań statutowych | NIE |
Materiał konferencyjny: | NIE |
Publikacja OA: | NIE |
Abstrakty: | angielski |