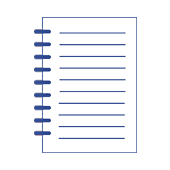
Fast bearing fault diagnosis of rolling element using Lévy Moth-Flame optimization algorithm and Naive Bayes
Artykuł w czasopiśmie
MNiSW
140
Lista 2021
Status: | |
Autorzy: | Sun Shuang, Przystupa Krzysztof, Wei Ming, Yu Han, Ye Zhiwei, Kochan Orest |
Dyscypliny: | |
Aby zobaczyć szczegóły należy się zalogować. | |
Rok wydania: | 2020 |
Wersja dokumentu: | Drukowana | Elektroniczna |
Język: | angielski |
Numer czasopisma: | 4 |
Wolumen/Tom: | 22 |
Strony: | 730 - 740 |
Impact Factor: | 2,176 |
Web of Science® Times Cited: | 39 |
Scopus® Cytowania: | 47 |
Bazy: | Web of Science | Scopus | BazTech | Index Copernicus |
Efekt badań statutowych | NIE |
Materiał konferencyjny: | NIE |
Publikacja OA: | TAK |
Licencja: | |
Sposób udostępnienia: | Otwarte czasopismo |
Wersja tekstu: | Ostateczna wersja opublikowana |
Czas opublikowania: | W momencie opublikowania |
Data opublikowania w OA: | 31 grudnia 2020 |
Abstrakty: | angielski |
Fault diagnosis is part of the maintenance system, which can reduce maintenance costs, increase productivity, and ensure the reliability of the machine system. In the fault diagnosis system, the analysis and extraction of fault signal characteristics are very important, which directly affects the accuracy of fault diagnosis. In the paper, a fast bearing fault diagnosis method based on the ensemble empirical mode decomposition (EEMD), the moth-flame optimization algorithm based on Lévy flight (LMFO) and the naive Bayes (NB) is proposed, which combines traditional pattern recognition methods meta-heuristic search can overcome the difficulty of selecting classifier parameters while solving small sample classification under reasonable time cost. The article uses a typical rolling bearing system to test the ac-tual performance of the method. Meanwhile, in comparison with the known algorithms and methods was also displayed in detail. The results manifest the efficiency and accuracy of signal sparse representation and fault type classification has been enhanced. |