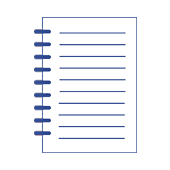
Application of Artificial Neural Networks for Yield Modeling of Winter Rapeseed Based on Combined Quantitative and Qualitative Data
Artykuł w czasopiśmie
MNiSW
100
Lista 2021
Status: | |
Autorzy: | Niedbała Gniewko, Piekutowska Magdalena, Weres Jerzy, Korzeniewicz Robert, Witaszek Kamil, Adamski Mariusz, Pilarski Krzysztof, Czechowska-Kosacka Aneta, Krysztofiak-Kaniewska Anna |
Dyscypliny: | |
Aby zobaczyć szczegóły należy się zalogować. | |
Rok wydania: | 2019 |
Wersja dokumentu: | Drukowana | Elektroniczna |
Język: | angielski |
Numer czasopisma: | 12 |
Wolumen/Tom: | 9 |
Numer artykułu: | 781 |
Strony: | 1 - 11 |
Impact Factor: | 2,603 |
Web of Science® Times Cited: | 29 |
Scopus® Cytowania: | 32 |
Bazy: | Web of Science | Scopus |
Efekt badań statutowych | NIE |
Materiał konferencyjny: | NIE |
Publikacja OA: | TAK |
Licencja: | |
Sposób udostępnienia: | Witryna wydawcy |
Wersja tekstu: | Ostateczna wersja opublikowana |
Czas opublikowania: | W momencie opublikowania |
Data opublikowania w OA: | 20 listopada 2019 |
Abstrakty: | angielski |
Rapeseed is considered as one of the most important oilseed crops in the world. Vegetable oil obtained from rapeseed is a valuable raw material for the food and energy industry as well as for industrial applications. Compared to other vegetable oils, it has a lower concentration of saturated fatty acids (5%–10%), a higher content of monounsaturated fatty acids (44%–75%), and a moderate content of alpha-linolenic acid (9%–13%). Overall, rapeseed is grown in all continents on an industrial scale, so there is a growing need to predict yield before harvest. A combination of quantitative and qualitative data were used in this work in order to build three independent prediction models, on the basis of which yield simulations were carried out. Empirical data collected during field tests carried out in 2008–2015 were used to build three models, QQWR15_4, QQWR31_5, and QQWR30_6. Each model was composed of a different number of independent variables, ranging from 21 to 27. The lowest MAPE (mean absolute percentage error) yield prediction error corresponded to QQWR31_5, it was 6.88%, and the coefficient of determination R2 was 0.69. As a result of the sensitivity analysis of the neural network, the most important independent variable influencing the final rapeseed yield was indicated, and for all the analyzed models it was “The kind of sowing date in the previous year” (KSD_PY). |