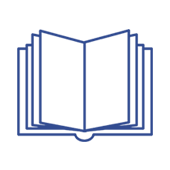
Convolutional Neural Networks with Reusable Full-Dimension-Long Layers for Feature Selection and Classification of Motor Imagery in EEG Signals
Fragment książki (Materiały konferencyjne)
MNiSW
70
konferencja
Status: | |
Autorzy: | Tokovarov Mikhail |
Dyscypliny: | |
Aby zobaczyć szczegóły należy się zalogować. | |
Wersja dokumentu: | Drukowana | Elektroniczna |
Język: | angielski |
Strony: | 79 - 91 |
Web of Science® Times Cited: | 4 |
Scopus® Cytowania: | 4 |
Bazy: | Web of Science | Scopus |
Efekt badań statutowych | NIE |
Materiał konferencyjny: | TAK |
Nazwa konferencji: | 29th International Conference on Artificial Neural Networks |
Skrócona nazwa konferencji: | ICANN 2020 |
URL serii konferencji: | LINK |
Termin konferencji: | 15 września 2020 do 18 września 2020 |
Miasto konferencji: | Bratislava |
Państwo konferencji: | SŁOWACJA |
Publikacja OA: | NIE |
Abstrakty: | angielski |
In the present article the author addresses the task of classification of motor imagery in EEG signals by proposing innovative architecture of neural network. Despite all the successes of deep learning, neural networks of significant depth could not ensure better performance compared to shallow architectures. The approach presented in the article employs this idea, making use of yet shallower, but productive architecture. The main idea of the proposed architecture is based on three points: full-dimension-long ‘valid’ convolutions, dense connections - combination of layer’s input and output and layer reuse. Another aspect addressed in the paper is related to interpretable machine learning. Interpretability is extremely important in medicine, where decisions must be taken on the basis of solid arguments and clear reasons. Being shallow, the architecture could be used for feature selection by interpreting the layers’ weights, which allows understanding of the knowledge about the data cumulated in the network’s layers. The approach, based on a fuzzy measure, allows using Choquet integral to aggregate the knowledge generated in the layer weights and understanding which features (EEG electrodes) provide the most essential information. The approach allows lowering feature number from 64 to 14 with an insignificant drop of accuracy (less than a percentage point). |