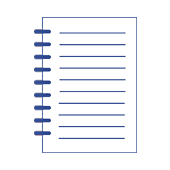
Estimation of the Path-Loss Exponent by Bayesian Filtering Method
Artykuł w czasopiśmie
MNiSW
100
Lista 2021
Status: | |
Autorzy: | Wójcicki Piotr, Zientarski Tomasz, Charytanowicz Małgorzata, Łukasik Edyta |
Dyscypliny: | |
Aby zobaczyć szczegóły należy się zalogować. | |
Rok wydania: | 2021 |
Wersja dokumentu: | Drukowana | Elektroniczna |
Język: | angielski |
Numer czasopisma: | 6 |
Wolumen/Tom: | 21 |
Numer artykułu: | 1934 |
Strony: | 1 - 11 |
Web of Science® Times Cited: | 13 |
Scopus® Cytowania: | 14 |
Bazy: | Web of Science | Scopus |
Efekt badań statutowych | NIE |
Materiał konferencyjny: | NIE |
Publikacja OA: | TAK |
Licencja: | |
Sposób udostępnienia: | Witryna wydawcy |
Wersja tekstu: | Ostateczna wersja opublikowana |
Czas opublikowania: | W momencie opublikowania |
Data opublikowania w OA: | 10 marca 2021 |
Abstrakty: | angielski |
Regarding wireless sensor network parameter estimation of the propagation model is a most important issue. Variations of the received signal strength indicator (RSSI) parameter are a fundamental problem of a system based on signal strength. In the present paper, we propose an algorithm based on Bayesian filtering techniques for estimating the path-loss exponent of the log-normal shadowing propagation model for outdoor RSSI measurements. Furthermore, in a series of experiments, we will demonstrate the usefulness of the particle filter for estimating the RSSI data. The stability of this algorithm and the differences in determined path-loss exponent for both method were also analysed. The proposed method of dynamic estimation results in significant improvements of the accuracy of RSSI values when compared with the experimental measurements. It should be emphasised that the path-loss exponent mainly depends on the RSSI data. Our results also indicate that increasing the number of inserted particles does not significantly raise the quality of the estimated parameters. |