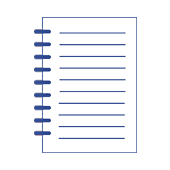
Online Measurement Error Detection for the ElectronicTransformer in a Smart Grid
Artykuł w czasopiśmie
MNiSW
140
Lista 2021
Status: | |
Autorzy: | Xiong Gu, Przystupa Krzysztof, Teng Yao, Xue Wang, Huan Wang, Feng Zhou, Qiong Xiang, Wang Chunzhi, Skowron Mikołaj, Kochan Orest, Beshley Mykola |
Dyscypliny: | |
Aby zobaczyć szczegóły należy się zalogować. | |
Rok wydania: | 2021 |
Wersja dokumentu: | Drukowana | Elektroniczna |
Język: | angielski |
Numer czasopisma: | 12 |
Wolumen/Tom: | 14 |
Numer artykułu: | 3551 |
Strony: | 1 - 18 |
Impact Factor: | 3,252 |
Web of Science® Times Cited: | 8 |
Scopus® Cytowania: | 16 |
Bazy: | Web of Science | Scopus |
Efekt badań statutowych | NIE |
Finansowanie: | This work is funded by Research on Key Technologies of online monitoring and evaluation of metering performance of 110~500 kV power transformer (JLW17201900078) and the National Natural Science Foundation of China under Grant No. 61772180. This work was financed as part of the Lublin University of Technology project-Regional Excellence Initiative, co-financed by the Ministry of Science and Higher Education (contract No. 030/RID/2018/19) and project: FD-20/EE- 2/801. |
Materiał konferencyjny: | NIE |
Publikacja OA: | TAK |
Licencja: | |
Sposób udostępnienia: | Witryna wydawcy |
Wersja tekstu: | Ostateczna wersja opublikowana |
Czas opublikowania: | W momencie opublikowania |
Data opublikowania w OA: | 15 czerwca 2021 |
Abstrakty: | angielski |
With the development of smart power grids, electronic transformers have been widely used to monitor the online status of power grids. However, electronic transformers have the drawback of poor long-term stability, leading to a requirement for frequent measurement. Aiming to monitor the online status frequently and conveniently, we proposed an attention mechanism-optimized Seq2Seq network to predict the error state of transformers, which combines an attention mechanism, Seq2Seq network, and bidirectional long short-term memory networks to mine the sequential information from online monitoring data of electronic transformers. We implemented the proposed method on the monitoring data of electronic transformers in a certain electric field. Experiments showed that our proposed attention mechanism-optimized Seq2Seq network has high accuracy in the aspect of error prediction. |