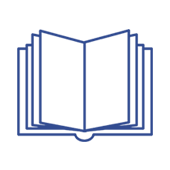
The Use of Principal Component Analysis and Logistic Regression for Cutter State Identification
Fragment książki (Rozdział monografii pokonferencyjnej)
MNiSW
20
Poziom I
Status: | |
Autorzy: | Kozłowski Edward, Mazurkiewicz Dariusz, Sęp Jarosław, Żabiński Tomasz |
Dyscypliny: | |
Aby zobaczyć szczegóły należy się zalogować. | |
Wersja dokumentu: | Drukowana | Elektroniczna |
Język: | angielski |
Strony: | 396 - 405 |
Web of Science® Times Cited: | 0 |
Scopus® Cytowania: | 6 |
Bazy: | Web of Science | Scopus |
Efekt badań statutowych | NIE |
Materiał konferencyjny: | TAK |
Nazwa konferencji: | First International Scientific Conference "Innovations in Industrial Engineering" |
Skrócona nazwa konferencji: | ICIENG 2021 ; ICIE 2021 |
URL serii konferencji: | LINK |
Termin konferencji: | 28 czerwca 2021 do 30 czerwca 2021 |
Miasto konferencji: | Guimarães |
Państwo konferencji: | PORTUGALIA |
Publikacja OA: | NIE |
Abstrakty: | angielski |
It is well known that due to Industry 4.0 requirements and challenges, future research directions in production engineering will focus on the creation of intelligent sensors and their integration by means of intelligent platforms. Therefore, the key skill will be appropriate analysis and processing of signals recorded by these sensors, which may relate to manufacturing process parameters. The application of principal component analysis and logistic regression enables effective data processing. This has been shown using a real-world numerical example - the data related to cutter state identification based on signals generated during machining. This way, it has been proven, that the above methods may find practical application in condition monitoring systems. In particular, they may be highly helpful in real-time cutter state identification. |