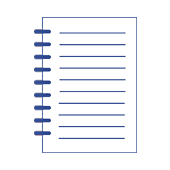
Mathematical modelling as an element of planning rail transport strategies
Artykuł w czasopiśmie
MNiSW
70
Lista 2021
Status: | |
Autorzy: | Borucka Anna, Mazurkiewicz Dariusz, Łagowska Eliza |
Dyscypliny: | |
Aby zobaczyć szczegóły należy się zalogować. | |
Rok wydania: | 2021 |
Wersja dokumentu: | Drukowana | Elektroniczna |
Język: | angielski |
Numer czasopisma: | 4 |
Wolumen/Tom: | 36 |
Strony: | 354 - 363 |
Impact Factor: | 1,455 |
Web of Science® Times Cited: | 6 |
Scopus® Cytowania: | 7 |
Bazy: | Web of Science | Scopus |
Efekt badań statutowych | NIE |
Materiał konferencyjny: | NIE |
Publikacja OA: | TAK |
Licencja: | |
Sposób udostępnienia: | Witryna wydawcy |
Wersja tekstu: | Ostateczna wersja opublikowana |
Czas opublikowania: | W momencie opublikowania |
Data opublikowania w OA: | 7 grudnia 2021 |
Abstrakty: | angielski |
Effective planning and optimization of rail transport operations depends on effective and reliable forecasting of demand. The results of transport performance forecasts usually differ from measured values because the mathematical models used are inadequate. In response to this applicative need, we report the results of a study whose goal was to de- velop, on the basis of historical data, an effective mathematical model of rail passenger transport performance that would allow to make reliable forecasts of future demand for this service. Several models dedicated to this type of empirical data were proposed and selection criteria were established. The models used in the study are: the seasonal naive model, the Ex- ponential Smoothing (ETS) model, the exponential smoothing state space model with Box–Cox transformation, ARMA errors, trigonometric trend and seasonal components (TBATS) model, and the AutoRegressive Integrated Moving Average (ARIMA) model. The proposed time series identification and forecasting methods are dedicated to the processing of time series data with trend and seasonality. Then, the best model was identified and its accuracy and effectiveness were assessed. It was noticed that investigated time series is characterized by strong seasonality and an upward trend. This information is important for planning a development strategy for rail passenger transport, because it shows that additional investments and engagement in the development of both transport infrastructure and superstructure are required to meet the existing demand. Finally, a forecast of transport performance in sequential periods of time was presented. Such forecast may sig- nificantly improve the system of scheduling train journeys and determining the level of demand for rolling stock depend- ing on the season and the annual rise in passenger numbers, increasing the effectiveness of management of rail transport. |