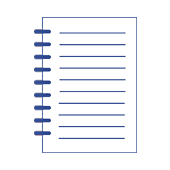
Forecasting short-term electric load using extreme learning machine with improved tree seed algorithm based on Lévy flight
Artykuł w czasopiśmie
MNiSW
140
Lista 2021
Status: | |
Autorzy: | Chen Xuan, Przystupa Krzysztof, Ye Zhiwei, Chen Feng, Wang Chunzhi, Liu Jinhang, Gao Rong, Wei Ming, Kochan Orest |
Dyscypliny: | |
Aby zobaczyć szczegóły należy się zalogować. | |
Rok wydania: | 2022 |
Wersja dokumentu: | Drukowana | Elektroniczna |
Język: | angielski |
Numer czasopisma: | 1 |
Wolumen/Tom: | 24 |
Strony: | 153 - 162 |
Impact Factor: | 2,5 |
Web of Science® Times Cited: | 9 |
Scopus® Cytowania: | 19 |
Bazy: | Web of Science | Scopus |
Efekt badań statutowych | NIE |
Finansowanie: | This research was funded by the National Natural Science Foundation of China under Grant No.61502155, 61772180, and the technological innovation project of Hubei Province 2019 No.2019AAA04, also funded by Fujian Provincial Key Laboratory of Data Intensive Computing and Key Laboratory of Intelligent Computing and Information Processing, Fujian No.BD201801. This work was financed in the framework of the project Lublin University of Technology - Regional Excellence Initiative, funded by thePolish Ministry of Science and Higher Education (contract no. 030/RID/2018/19). |
Materiał konferencyjny: | NIE |
Publikacja OA: | TAK |
Licencja: | |
Sposób udostępnienia: | Witryna wydawcy |
Wersja tekstu: | Ostateczna wersja opublikowana |
Czas opublikowania: | W momencie opublikowania |
Data opublikowania w OA: | 26 stycznia 2022 |
Abstrakty: | angielski |
In recent years, forecasting has received increasing attention since it provides an important basis for the effective operation of power systems. In this paper, a hybrid method, composed of kernel principal component analysis (KPCA), tree seed algorithm based on Lévy flight (LTSA) and extreme learning machine (ELM), is proposed for short-term load forecasting. Specifically, the randomly generated weights and biases of ELM have a significant impact on the stability of prediction results. Therefore, in order to solve this problem, LTSA is utilized to obtain the optimal parameters before the prediction process is executed by ELM, which is called LTSA-ELM. Meanwhile, the input data is extracted by KPCA considering the sparseness of the electric load data and used as the input of LTSA-ELM model. The proposed method is tested on the data from European network on intelligent technologies (EUNITE) and experimental results demonstrate the superiority of the proposed approaches compared to the other methods involved in the paper. |