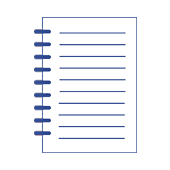
2D Geometric Surface Structure ANN Modeling after Milling of the AZ91D Magnesium Alloy
Artykuł w czasopiśmie
MNiSW
100
Lista 2021
Status: | |
Autorzy: | Kulisz Monika, Zagórski Ireneusz, Józwik Jerzy |
Dyscypliny: | |
Aby zobaczyć szczegóły należy się zalogować. | |
Rok wydania: | 2022 |
Wersja dokumentu: | Drukowana | Elektroniczna |
Język: | angielski |
Numer czasopisma: | 2 |
Wolumen/Tom: | 16 |
Strony: | 131 - 140 |
Impact Factor: | 1,1 |
Web of Science® Times Cited: | 2 |
Scopus® Cytowania: | 5 |
Bazy: | Web of Science | Scopus | BazTech |
Efekt badań statutowych | NIE |
Finansowanie: | This work was financed from the funds of the Ministry of Education and Science by Agreement No. DNK/SP/513880/2021 of 22 December 2021, the project “14th School of Machining and the 43rd Scientific School of Abrasive Machining”, under the programme “Perfect Science”. |
Materiał konferencyjny: | NIE |
Publikacja OA: | TAK |
Licencja: | |
Sposób udostępnienia: | Otwarte czasopismo |
Wersja tekstu: | Ostateczna wersja opublikowana |
Czas opublikowania: | W momencie opublikowania |
Data opublikowania w OA: | 3 marca 2022 |
Abstrakty: | angielski |
The paper presents the results of modeling 2D surface roughness parameters in milling by means of an artificial neural network (ANN). The AZ91D magnesium alloy was used. A HSS milling cutter was employed in the research. The main aim of the study was to obtain the lowest possible surface roughness (good quality) using a commonly available HSS cutter. The results of the research work were presented in the form of bar charts, surface charts and graphs depicting the quality of artificial neural networks. The conducted research shows that it is possible to carry out the machining processes that enable obtaining an average surface quality (defined by roughness parameters Ra, Rz, RSm, Rsk). The Ra, Rz, RSm parameters increase along with the machining parameters (fz, ap), as expected. The Rsk parameter takes (in most cases) negative values, which may indicate a surface with more intense friction and indicative of flat-topped distribution. On the other hand, the results of modeling the selected parameters – Ra, Rz, RSm – with the use of artificial neural networks allow concluding that the obtained network models show satisfactory predictive ability (R = 0.99), and thus are an appropriate tool for the prediction of surface roughness parameters. |