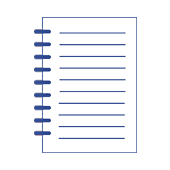
Forecasting of Power Output of a PVPS Based on Meteorological Data Using RNN Approaches
Artykuł w czasopiśmie
MNiSW
100
Lista 2021
Status: | |
Autorzy: | Beigi Mohsen, Harchegani Hossein Beigi, Torki Mehdi, Kaveh Mohammad, Szymanek Mariusz, Khalife Esmail, Dziwulski Jacek |
Dyscypliny: | |
Aby zobaczyć szczegóły należy się zalogować. | |
Rok wydania: | 2022 |
Wersja dokumentu: | Drukowana | Elektroniczna |
Język: | angielski |
Numer czasopisma: | 5 |
Wolumen/Tom: | 14 |
Numer artykułu: | 3104 |
Strony: | 1 - 12 |
Impact Factor: | 3,9 |
Web of Science® Times Cited: | 5 |
Scopus® Cytowania: | 7 |
Bazy: | Web of Science | Scopus |
Efekt badań statutowych | NIE |
Materiał konferencyjny: | NIE |
Publikacja OA: | TAK |
Licencja: | |
Sposób udostępnienia: | Witryna wydawcy |
Wersja tekstu: | Ostateczna wersja opublikowana |
Czas opublikowania: | W momencie opublikowania |
Data opublikowania w OA: | 7 marca 2022 |
Abstrakty: | angielski |
Artificial intelligence (AI) has become increasingly popular as a tool to model, identify, optimize, forecast, and control renewable energy systems. This work aimed to evaluate the capability of the artificial neural network (ANN) procedure to model and forecast solar power outputs of photovoltaic power systems (PVPSs) by using meteorological data. For this purpose, based on the literature review, important factors affecting energy generation in a PVPS were selected as inputs, and a recurrent neural network (RNN) architecture was established. After completing the trained network, the RNN capability was assessed to predict the energy output of the PVPS for days not included in the training database. The performance evaluation of the trained RNN revealed a regression value of 0.97774 for test data, whereas the RMSE and the mean actual output power for a sample day were 0.0248 MJ and 0.538 MJ, respectively. In addition to RMSE, an error histogram and regression plots obtained by MATLAB were employed to evaluate the network’s capability, and validation results represented a sufficient prediction accuracy of the trained RNN. |