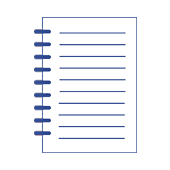
Application of Deep Convolutional Neural Networks in the Diagnosis of Osteoporosis
Artykuł w czasopiśmie
MNiSW
100
Lista 2021
Status: | |
Autorzy: | Dzierżak Róża, Omiotek Zbigniew |
Dyscypliny: | |
Aby zobaczyć szczegóły należy się zalogować. | |
Rok wydania: | 2022 |
Wersja dokumentu: | Drukowana | Elektroniczna |
Język: | angielski |
Numer czasopisma: | 21 |
Wolumen/Tom: | 22 |
Numer artykułu: | 8189 |
Strony: | 1 - 18 |
Impact Factor: | 3,9 |
Web of Science® Times Cited: | 7 |
Scopus® Cytowania: | 10 |
Bazy: | Web of Science | Scopus |
Efekt badań statutowych | NIE |
Finansowanie: | This research was funded by the Ministry of Education and Science—Poland, grant number FD-20/EE-2/302 and FD-20/EE-2/315. |
Materiał konferencyjny: | NIE |
Publikacja OA: | TAK |
Licencja: | |
Sposób udostępnienia: | Witryna wydawcy |
Wersja tekstu: | Ostateczna wersja opublikowana |
Czas opublikowania: | W momencie opublikowania |
Data opublikowania w OA: | 26 października 2022 |
Abstrakty: | angielski |
The aim of this study was to assess the possibility of using deep convolutional neural networks (DCNNs) to develop an effective method for diagnosing osteoporosis based on CT images of the spine. The research material included the CT images of L1 spongy tissue belonging to 100 patients (50 healthy and 50 diagnosed with osteoporosis). Six pre-trained DCNN architectures with different topological depths (VGG16, VGG19, MobileNetV2, Xception, ResNet50, and InceptionResNetV2) were used in the study. The best results were obtained for the VGG16 model characterised by the lowest topological depth (ACC = 95%, TPR = 96%, and TNR = 94%). A specific challenge during the study was the relatively small (for deep learning) number of observations (400 images). This problem was solved using DCNN models pre-trained on a large dataset and a data augmentation technique. The obtained results allow us to conclude that the transfer learning technique yields satisfactory results during the construction of deep models for the diagnosis of osteoporosis based on small datasets of CT images of the spine. |