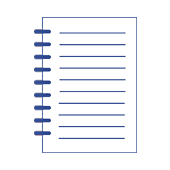
A neural Network approach for reconstructing in-cylinder pressure from engine vibration data
Artykuł w czasopiśmie
MNiSW
40
Lista 2021
Status: | |
Autorzy: | Nguyen Hoang Khac, Modabberian Amin, Zenger Kai, Lendormy Éric, Mikulski Maciej, Hunicz Jacek |
Dyscypliny: | |
Aby zobaczyć szczegóły należy się zalogować. | |
Rok wydania: | 2022 |
Wersja dokumentu: | Drukowana | Elektroniczna |
Język: | angielski |
Numer czasopisma: | 01-1038 |
Strony: | 1 - 12 |
Scopus® Cytowania: | 5 |
Bazy: | Scopus |
Efekt badań statutowych | NIE |
Materiał konferencyjny: | TAK |
Nazwa konferencji: | SAE 2022 Powertrains, Fuels & Lubricants Conference & Exhibition |
Skrócona nazwa konferencji: | SAE 2022 |
URL serii konferencji: | LINK |
Termin konferencji: | 6 września 2022 do 8 września 2022 |
Miasto konferencji: | Kraków |
Państwo konferencji: | POLSKA |
Publikacja OA: | NIE |
Abstrakty: | angielski |
In this work neural network models are used to reconstruct in-cylinder pressure from a vibration signal measured from the engine surface by a low-cost accelerometer. Using accelerometers to capture engine combustion is a cost-effective approach due to their low price and flexibility. The paper describes a virtual sensor that re-constructs the in-cylinder pressure and some of its key parameters by using the engine vibration data as input. The vibration and cylinder pressure data have been processed before the neural network model training. Additionally, the correlation between the vibration and in-cylinder pressure data is analyzed to show that the vibration signal is a good input to model the cylinder pressure.The approach is validated on a RON95 single cylinder research engine realizing homogeneous charge compression ignition (HCCI). The experimental matrix covers multiple load/rpm steady-state operating points with different start of injection and lambda setpoints. A radial basis function (RBF) neural network model was first trained with a series of two operating points at low loads with data of 1000 consecutive combustion cycles, to build the needed nonlinear mapping. The results show that the developed neural network model is capable of reconstructing in-cylinder pressure at low loads with good accuracy. The error for combustion parameter such as maximum cylinder pressure did not exceed 5%. The approach is further validated with another series of operating points consisting of both low loads and high loads. However, the results in this case deteriorated. Changing the neural network model to generalized regression (GR) improved the in-cylinder pressure reconstruction quality. The performance of the models was also considered in terms of combustion parameters, such as maximum pressure and mass burned fraction. The paper concludes that vibration signal carries sufficient information to estimate combustion parameters independently on the engine platform or combustion concept. |