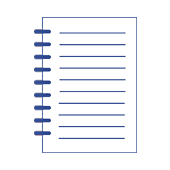
Identification of surface defects using deep and transfer learning
Artykuł w czasopiśmie
MNiSW
40
Lista 2021
Status: | |
Autorzy: | Kłosowski Grzegorz, Kulisz Monika |
Dyscypliny: | |
Aby zobaczyć szczegóły należy się zalogować. | |
Rok wydania: | 2022 |
Wersja dokumentu: | Drukowana | Elektroniczna |
Język: | angielski |
Wolumen/Tom: | 2408 |
Numer artykułu: | 012028 |
Strony: | 1 - 8 |
Scopus® Cytowania: | 1 |
Bazy: | Scopus |
Efekt badań statutowych | NIE |
Materiał konferencyjny: | TAK |
Nazwa konferencji: | XXX Sympozjum Środowiskowe PTZE Zastosowania Elektromagnetyzmu we Współczesnej Inżynierii i Medycynie |
Skrócona nazwa konferencji: | XXX PTZE |
URL serii konferencji: | LINK |
Termin konferencji: | 12 września 2021 do 15 września 2021 |
Miasto konferencji: | Jastarnia |
Państwo konferencji: | POLSKA |
Publikacja OA: | TAK |
Licencja: | |
Sposób udostępnienia: | Witryna wydawcy |
Wersja tekstu: | Ostateczna wersja opublikowana |
Czas opublikowania: | W momencie opublikowania |
Data opublikowania w OA: | 23 grudnia 2022 |
Abstrakty: | angielski |
The purpose of this study was to employ a previously trained (pre-trained) convolutional neural network called Resnet101 in conjunction with deep machine learning approaches in order to construct an algorithm for classifying cracks in the photos that were evaluated. Adjustments were made to the ultimate layer, which resulted in the fully connected layer being altered. Specifically, the basic 1000-output fully connected layer in Resnet101 was replaced with a binary-classification layer, which consisted of two categories: an image with cracks and an image without cracks. In this study, we investigate whether or not it is possible to use deep neural networks to accomplish the rapid and entirely automated detection of flaws by utilizing analyzed photographs as the data source. The research that was done led to the discovery that a pre-trained convolutional neural network that makes use of support vector machines to train a fully connected layer is quite an efficient option, and that the acquired forecasting algorithm allows the categorization of faults with extremely good accuracy. The proposed classification algorithm is 99 percent efficient. In material inspection tasks, this idea can be used to find cracks and other flaws in the material, such as those that could be found in a number of public structures like buildings, roads, and bridges. |