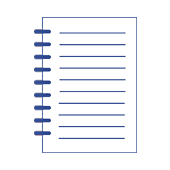
Interpretable Machine Learning for Prediction of Post-Fire Self-Healing of Concrete
Artykuł w czasopiśmie
MNiSW
140
Lista 2023
Status: | |
Autorzy: | Rajczakowska Magdalena, Szeląg Maciej, Habermehl-Cwirzen Karin, Hedlund Hans, Cwirzen Andrzej |
Dyscypliny: | |
Aby zobaczyć szczegóły należy się zalogować. | |
Rok wydania: | 2023 |
Wersja dokumentu: | Elektroniczna |
Język: | angielski |
Numer czasopisma: | 3 |
Wolumen/Tom: | 16 |
Numer artykułu: | 1273 |
Strony: | 1 - 29 |
Impact Factor: | 3,1 |
Web of Science® Times Cited: | 13 |
Scopus® Cytowania: | 13 |
Bazy: | Web of Science | Scopus | ADS (Astrophysics Data System) BibCnrs CABI CAPlus / SciFinder CNKI DOAJ EBSCO Ei Compendex Gale Inspec PubMed PMC OpenAIRE OSTI (U.S. Department of Energy) PATENTSCOPE ProQuest SafetyLit SCIE |
Efekt badań statutowych | NIE |
Finansowanie: | This project has received support from the Swedish Transport Administration (Trafikverket), Skanska AB, and the Development Fund of the Swedish Construction Industry (SBUF). |
Materiał konferencyjny: | NIE |
Publikacja OA: | TAK |
Licencja: | |
Sposób udostępnienia: | Witryna wydawcy |
Wersja tekstu: | Ostateczna wersja opublikowana |
Czas opublikowania: | W momencie opublikowania |
Data opublikowania w OA: | 2 lutego 2023 |
Abstrakty: | angielski |
Developing accurate and interpretable models to forecast concrete’s self-healing behavior is of interest to material engineers, scientists, and civil engineering contractors. Machine learning (ML) and artificial intelligence are powerful tools that allow constructing high-precision predictions, yet often considered “black box” methods due to their complexity. Those approaches are commonly used for the modeling of mechanical properties of concrete with exceptional accuracy; however, there are few studies dealing with the application of ML for the self-healing of cementitious materials. This paper proposes a pioneering study on the utilization of ML for predicting post-fire self-healing of concrete. A large database is constructed based on the literature studies. Twelve input variables are analyzed: w/c, age of concrete, amount of cement, fine aggregate, coarse aggregate, peak loading temperature, duration of peak loading temperature, cooling regime, duration of cooling, curing regime, duration of curing, and specimen volume. The output of the model is the compressive strength recovery, being one of the self-healing efficiency indicators. Four ML methods are optimized and compared based on their performance error: Support Vector Machines (SVM), Regression Trees (RT), Artificial Neural Networks (ANN), and Ensemble of Regression Trees (ET). Monte Carlo analysis is conducted to verify the stability of the selected model. All ML approaches demonstrate satisfying precision, twice as good as linear regression. The ET model is found to be the most optimal with the highest prediction accuracy and sufficient robustness. Model interpretation is performed using Partial Dependence Plots and Individual Conditional Expectation Plots. Temperature, curing regime, and amounts of aggregates are identified as the most significant predictors. |