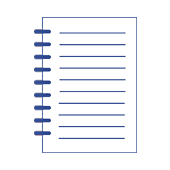
Social Recommendation Algorithm Based on Self-Supervised Hypergraph Attention
Artykuł w czasopiśmie
MNiSW
140
Lista 2023
Status: | |
Autorzy: | Xu Xiangdong, Przystupa Krzysztof, Kochan Orest |
Dyscypliny: | |
Aby zobaczyć szczegóły należy się zalogować. | |
Rok wydania: | 2023 |
Wersja dokumentu: | Drukowana | Elektroniczna |
Język: | angielski |
Numer czasopisma: | 4 |
Wolumen/Tom: | 12 |
Numer artykułu: | 906 |
Strony: | 1 - 19 |
Impact Factor: | 2,6 |
Web of Science® Times Cited: | 0 |
Scopus® Cytowania: | 7 |
Bazy: | Web of Science | Scopus |
Efekt badań statutowych | NIE |
Finansowanie: | This work was financed as part of the Lublin University of Technology project: FD-20/EE-2/801 and project: FD-20/IM-5/087. |
Materiał konferencyjny: | NIE |
Publikacja OA: | TAK |
Licencja: | |
Sposób udostępnienia: | Witryna wydawcy |
Wersja tekstu: | Ostateczna wersja opublikowana |
Czas opublikowania: | W momencie opublikowania |
Data opublikowania w OA: | 10 lutego 2023 |
Abstrakty: | angielski |
Social network information has been widely applied to traditional recommendations that have received significant attention in recent years. Most existing social recommendation models tend to use pairwise relationships to explore potential user preferences, but overlook the complexity of real-life interactions between users and the fact that user relationships may be higher order. These approaches also ignore the dynamic nature of friend influence, which leads the models to treat different friend influences equally in different ways. To address this, we propose a social recommendation algorithm that incorporates graph embedding and higher-order mutual information maximization based on the consideration of social consistency. Specifically, we use the graph attention model to build higher-order information among users for deeper mining of their behavioral patterns on the one hand; while on the other hand, it models user embedding based on the principle of social consistency to finally achieve finer-grained inference of user interests. In addition, to alleviate the problem of losing its own hierarchical information after fusing different levels of hypergraphs, we use self-supervised learning to construct auxiliary branches that fully enhance the rich information in the hypergraph. Experimental results conducted on two publicly available datasets show that the proposed model outperforms state-of-the-art methods. |