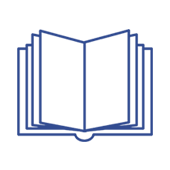
Novel Approach to Prognostics and Health Management to Combine Reliability and Process Optimisation
Fragment książki (Rozdział w monografii)
MNiSW
20
Poziom I
Status: | |
Autorzy: | Mazurkiewicz Dariusz, Ren Yi, Qian Cheng |
Dyscypliny: | |
Aby zobaczyć szczegóły należy się zalogować. | |
Wersja dokumentu: | Drukowana | Elektroniczna |
Język: | angielski |
Strony: | 559 - 580 |
Scopus® Cytowania: | 2 |
Bazy: | Scopus |
Efekt badań statutowych | NIE |
Finansowanie: | This work was supported by the individual research grant FD-20/IM-5/072/2022, of the scientific discipline Mechanical Engineering, Lublin University of Technology, Poland. This work was prepared within the project PM/SP/0063/2021/1 titled “Innovative measurement technologies supported by digital data processing algorithms for improved processes and products”, financed by the Ministry of Education and Science (Poland) as a part of the Polish Metrology Programme. This work was partially prepared under the project “Digital Twin Based Fault Prediction and Maintenance Method for Intelligent Instruments”—Grant No. G2021177009L under the framework of the “High-end International Visiting Professor Project” through long distance collaboration. |
Materiał konferencyjny: | NIE |
Publikacja OA: | NIE |
Abstrakty: | angielski |
Prognostics and Health Management (PHM) supports users with an integrated view of the health of any technical asset, and it consists of many different tasks based on data that are usually obtained from multisensory systems. The effective implementation of PHM does not, however, end with predicting remaining useful life (RUL). PHM has untapped potential to go beyond failure prediction and support of optimal maintenance actions and scheduling, along with logistics decisions. Both data captured by reliability systems and standard production data are generally used separately for different purposes. For higher effectiveness, these data have to be integrated in a combined approach. This can be achieved with the help of Digital Twin analytics that can support effective data use for parallel or combined purposes, such as classifying states, predicting failures or enhancing production efficiency. Furthermore, these seemingly independent concepts can be integrated into the same data collection approach. Previous studies have demonstrated that the afore-mentioned combined solution to classification and prediction challenges is yet only a standard approach to PHM, one that makes it possible to predict RUL, degradation track and optimal time to intervention. Consequently, a new solution is proposed, one that takes into consideration the possibility of intelligent and sustainable production in combination with online predictive maintenance and continuous process optimisation. The prediction of degradation and remaining useful life with the use of multisource data integration facilitates production process optimisation to gain additional use time. This, in turn, brings about incomparably greater financial effects than is the case with the traditional approach to PHM. |