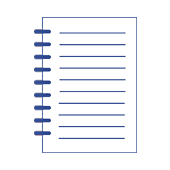
A Knowledge Graph Embedding Based Service Recommendation Method for Service-Based System Development
Artykuł w czasopiśmie
MNiSW
140
Lista 2023
Status: | |
Autorzy: | Xie Fang, Zhang Yiming, Przystupa Krzysztof, Kochan Orest |
Dyscypliny: | |
Aby zobaczyć szczegóły należy się zalogować. | |
Rok wydania: | 2023 |
Wersja dokumentu: | Drukowana | Elektroniczna |
Język: | angielski |
Numer czasopisma: | 13 |
Wolumen/Tom: | 12 |
Numer artykułu: | 2935 |
Strony: | 1 - 14 |
Impact Factor: | 2,6 |
Web of Science® Times Cited: | 2 |
Scopus® Cytowania: | 4 |
Bazy: | Web of Science | Scopus |
Efekt badań statutowych | NIE |
Finansowanie: | This work is supported by the Key Project of Hubei Education Department under Grant No. D20201402; the Natural Science Foundation of Hubei Province under Grant No. 2020CFB807; the Science Start-up Foundation for High-level Talents of HBUT under Grant No. 430100391 |
Materiał konferencyjny: | NIE |
Publikacja OA: | TAK |
Licencja: | |
Sposób udostępnienia: | Witryna wydawcy |
Wersja tekstu: | Ostateczna wersja opublikowana |
Czas opublikowania: | W momencie opublikowania |
Data opublikowania w OA: | 4 lipca 2023 |
Abstrakty: | angielski |
Web API is an efficient way for Service-based Software (SBS) development, and mashup is a key technology which merges several web services to deal with the increasing complexity of software requirements and expedite the service-based system development. The efficient service recommendation method is vital for the software development. However, the existing methods often suffer from data sparsity or cold start issues, which should lead to bad effects. Currently, this paper starts with SBS development, and proposes a service recommendation method based on knowledge graph embedding and collaborative filtering (CF) technology. In our model, we first construct a refined knowledge graph using SBS-service co-invocation record and SBS and service related information to mine the potential semantics relationship between SBS and service. Then, we learn the SBS and service entities in the knowledge graph. These heterogeneous entities (SBS and service, etc.) are embedded into the low-dimensional space through the representation learning algorithms of Word2vec and TransR, and the distances between SBS and service vectors are calculated. The input of recommendation model is SBS requirement (target SBS), the similarities functional SBS set is extracted from knowledge graph, which can relieve the cold start problem. Meanwhile, the recommendation model uses CF to recommend service to target SBS. Finally, this paper verifies the effectiveness of method on the real-word dataset. Compared with the several state-of-the-art methods, our method has the best service hit rate and ranking quality. |