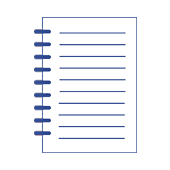
BSANet: High-Performance 3D Medical Image Segmentation
Artykuł w czasopiśmie
MNiSW
100
Lista 2023
Status: | |
Autorzy: | Huang Qi, Su Jun, Przystupa Krzysztof, Kochan Orest |
Dyscypliny: | |
Aby zobaczyć szczegóły należy się zalogować. | |
Rok wydania: | 2023 |
Wersja dokumentu: | Drukowana | Elektroniczna |
Język: | angielski |
Wolumen/Tom: | 11 |
Strony: | 79213 - 79223 |
Impact Factor: | 3,4 |
Web of Science® Times Cited: | 0 |
Scopus® Cytowania: | 0 |
Bazy: | Web of Science | Scopus |
Efekt badań statutowych | NIE |
Finansowanie: | This work was supported by the Artificial Intelligence Laboratory of Hubei University of Technology. |
Materiał konferencyjny: | NIE |
Publikacja OA: | TAK |
Licencja: | |
Sposób udostępnienia: | Otwarte czasopismo |
Wersja tekstu: | Ostateczna wersja opublikowana |
Czas opublikowania: | W momencie opublikowania |
Data opublikowania w OA: | 27 lipca 2023 |
Abstrakty: | angielski |
As a challenge in the field of smart medicine, medical picture segmentation gives important decisions and is the basis for future diagnosis by doctors. In the past decade, FCN-based network topologies have made amazing progress in the field. However, the limited perceptual capacity of convolutional kernels in FCN network topologies limits the network's ability to acquire a global field of view. We propose BSANet, a 3D medical image segmentation network based on self-focus and multi- scale information fusion with a high-performance feature extraction module. BSANet can help the network to extract deeper features by obtaining a larger range of perceptual capabilities by using its self- focus and multi-scale information aggregation pooling modules. Brain tumor segmentation dataset and multi-organ segmentation dataset are used to train and evaluate our model. BSANet produces excellent results with its high-performance feature extraction network with an attention module and multi-scale information fusion modul. |