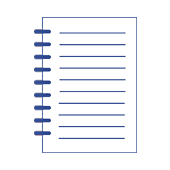
DCELANM-Net: Medical image segmentation based on dual channel efficient layer aggregation network with learner
Artykuł w czasopiśmie
MNiSW
70
Lista 2024
Status: | |
Autorzy: | Lu Chengzhun, Xia Zhangrun, Przystupa Krzysztof, Kochan Orest, Su Jun |
Dyscypliny: | |
Aby zobaczyć szczegóły należy się zalogować. | |
Rok wydania: | 2024 |
Wersja dokumentu: | Elektroniczna |
Język: | angielski |
Numer czasopisma: | 1 |
Wolumen/Tom: | 34 |
Numer artykułu: | e22960 |
Strony: | 1 - 14 |
Web of Science® Times Cited: | 0 |
Scopus® Cytowania: | 0 |
Bazy: | Web of Science | Scopus |
Efekt badań statutowych | NIE |
Materiał konferencyjny: | NIE |
Publikacja OA: | NIE |
Abstrakty: | angielski |
Segmenting medical images is a principal component of computer vision. The UNet model framework has taken over as the standard framework for this activity across a wide range of medical picture segmentation applications. Due to convolutional neural networks (CNNs) convolution operation limitations, the model's global modeling ability is not absolutely perfect. Moreover, a single convolution operation cannot gather feature information at various scales, which will have an impact on the quality of the global feature extraction as well as the localization of local details. The DCELANM-Net structure, which this article offers, is a model that ingeniously combines a Dual Channel Efficient Layer Aggregation Network (DCELAN) and a Micro Masked Autoencoder (Micro-MAE). On the one hand, for the DCELAN, the features are more effectively fitted by deepening the network structure, which in turn can successfully learn and fuse the features, helping to locate the local feature information more accurately; and the utilization of each layer of channels is more effectively improved by widening the network structure and residual connections. We adopt Micro-MAE as the learner of the model. In addition to being straightforward in its methodology, it also includes a self-supervised learning method, which has the benefit of being incredibly scalable for the model. This scalable method enables the generalization of high-volume models, and the models can show good scaling behavior. It is also shown that Micro-MAE is a powerful and adaptable learner that we can incorporate it into our network design to improve the model's accuracy and stability for tasks involving medical picture segmentation. Superior metrics and good generalization are demonstrated by DCELANM-Net on the datasets Kvasir-SEG and CVC-ClinicDB. In the experiments, we set DCELANM-S and DCELANM-L to represent different sizes of the model, and since DCELANM-L has the best performance, DCELANM-L is determined as the base model for all experiments, called DCELANM. |