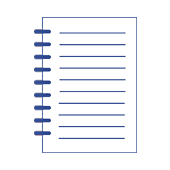
Sensitivity analysis of crack propagation in pavement bituminous layered structures using a hybrid system integrating Artificial Neural Networks and Finite Element Method
Artykuł w czasopiśmie
MNiSW
30
Lista A
Status: | |
Autorzy: | Gajewski Jakub, Sadowski Tomasz |
Rok wydania: | 2014 |
Wersja dokumentu: | Drukowana | Elektroniczna |
Język: | angielski |
Wolumen/Tom: | 82 |
Strony: | 114 - 117 |
Impact Factor: | 2,131 |
Web of Science® Times Cited: | 66 |
Scopus® Cytowania: | 84 |
Bazy: | Web of Science | Scopus | Web of Science | Scopus |
Efekt badań statutowych | NIE |
Materiał konferencyjny: | NIE |
Publikacja OA: | NIE |
Abstrakty: | angielski |
The paper presents results of sensitivity analysis to crack propagation of a pavement bituminous layered structure using the Finite Element Method (FEM) and Artificial Neural Networks (ANN). The performed preliminary study determined stresses and displacements in the pavement layer system. The pavement structure consisting of asphalt layers, a base layer, subbase and subgrade layers was analyzed as a 2D finite element model using the ABAQUS computer software. The second method, i.e. the extend Finite Element Method was applied, to simulate cracking process of the bituminous layer of a road surface. The pavement model was subjected to static load. Both linear and non-linear material properties of the pavement layers were considered to discuss crack propagation sensitivity in the pavement layers. A hybrid system integrating Artificial Neural Networks (ANN) and FEM was considered to model the material of asphalt layers in flexible pavements. The tests for Radial Basis Function (RBF) and Multi-Layer Perceptron (MLP) networks were carried out. In the formulated model the ANN requires inputs such as: layers thickness, load value and the Young’s moduli of each layer creating the pavement. The data for the ANN were obtained from Finite Element Method analysis. The aim of the network learning process as non-destructive testing was to evaluate the pavement material behavior and estimation of the crack propagation sensitivity. The main conclusion is that cracking considerably increases with a decrease in the thickness of bituminous layer B2. The thickness of the asphalt layer B1 has much less considerable effect on the cracking of the subgrade layer. |