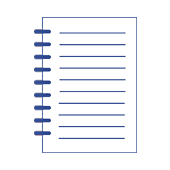
Sequential algorithm of building the regression-classification model for total nitrogen simulation: application of machine learning
Artykuł w czasopiśmie
MNiSW
100
Lista 2023
Status: | |
Autorzy: | Barbusiński Krzysztof, Szeląg Bartosz, Białek Anita, Łazuka Ewa, Popławska Emilia, Szulżyk-Cieplak Joanna, Babko Roman, Łagód Grzegorz |
Dyscypliny: | |
Rok wydania: | 2023 |
Wersja dokumentu: | Drukowana | Elektroniczna |
Język: | angielski |
Wolumen/Tom: | 301 |
Strony: | 106 - 114 |
Impact Factor: | 1,0 |
Web of Science® Times Cited: | 0 |
Scopus® Cytowania: | 0 |
Bazy: | Web of Science | Scopus |
Efekt badań statutowych | NIE |
Materiał konferencyjny: | NIE |
Publikacja OA: | NIE |
Abstrakty: | angielski |