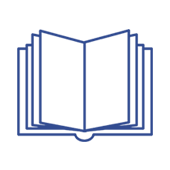
Reinforcement of self-regulated learning in the it laboratory
Fragment książki (Rozdział monografii pokonferencyjnej)
MNiSW
5
spoza wykazu
Status: | |
Autorzy: | Przyłucki Sławomir, Czerwińska Magdalena |
Dyscypliny: | |
Aby zobaczyć szczegóły należy się zalogować. | |
Wersja dokumentu: | Elektroniczna |
Język: | angielski |
Strony: | 4989 - 4998 |
Efekt badań statutowych | NIE |
Materiał konferencyjny: | TAK |
Nazwa konferencji: | 16th annual International Conference of Education, Research and Innovation : conference proceedings |
Skrócona nazwa konferencji: | ICERI 2023 |
URL serii konferencji: | LINK |
Termin konferencji: | 13 listopada 2023 do 15 listopada 2023 |
Miasto konferencji: | Seville |
Państwo konferencji: | HISZPANIA |
Publikacja OA: | NIE |
Abstrakty: | angielski |
he article presents an analysis of the results of using self-regulated learning paradigms in the education process at technical universities in the last four years. The analysis of scientific publications shows that this paradigm is particularly important in teaching at the academic level. This is mainly due to the need for students to switch from teaching schemes commonly used in secondary schools, where the teacher plays a leading role, to university rules. The results of published research as well as the authors' experience clearly indicate that in many cases this is a significant barrier for many students. The article proposes a model based on the paradigm of self-regulated learning, which was called Reinforcement Self-Regulated Learning (RSR Learning). It allows to significantly reduce the impact of the barriers described above and is an extension of the popular goal-oriented model, very often used in solutions based on self-regulated learning. The structure of the proposed RSR Learning model consists of three basic elements. The first is the database of laboratory projects (LPdb) and contains a scalable set of project descriptions related to a certain number of key issues taught in each thematic block of the laboratory. In this case, scaling consists in gradation of difficulty within individual key issues and gives the opportunity to choose the optimal substantive content of a given project. This allows for the adaptation of requirements in close connection with the current learning results of a given student. Each project has a precisely defined set of parameters that allow for an objective assessment and comparison of the student's achievements. These parameters define whether the goal has been achieved and how well, and at the same time they are the basis for determining the average effectiveness of teaching at a given stage of classes. The parameters are processed in the next element of the model, which is the evaluation block. It contains a set of parameterized rules (algorithms) that guarantee an objective but also flexible description (assessment) of the relationship between the learning results achieved by a particular student and the average results within his laboratory group and all groups simultaneously performing similar tasks. Therefore, it allows for effective and flexible control of the process of mutual competition. This block directly interacts with the third component of the model, the feedback block (reinforcement block). Its purpose is to link the obtained learning outcomes with the selection of the structure of subsequent projects built based on the LPdb database. It therefore serves as a feedback loop reinforcing the learning process. The structure and functioning of the model are described in detail in the first part of the article. Its main part contains an analysis of learning outcomes from the last four years on the example of a cloud computing laboratory. The data is compared with the teaching effects obtained using the classic methodology based on the goal-oriented model. Since distance learning methods were used in the years 2020-2021, the analysis also allows to assess the effectiveness of the proposed model in distance learning. The collected data come from both the first and second degree of academic education. Therefore, the presented analysis gives an answer to the question of how the complexity of the taught content affects the effectiveness of the proposed RSR Learning model. |