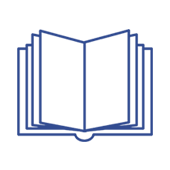
Explainable Ensemble Machine Learning for Wheat Grain Identification
Fragment książki (Materiały konferencyjne)
MNiSW
200
konferencja
Status: | |
Autorzy: | Charytanowicz Małgorzata |
Dyscypliny: | |
Aby zobaczyć szczegóły należy się zalogować. | |
Wersja dokumentu: | Drukowana | Elektroniczna |
Język: | angielski |
Strony: | 903 - 911 |
Web of Science® Times Cited: | 0 |
Scopus® Cytowania: | 0 |
Bazy: | Web of Science | Scopus | IEEE Xplore |
Efekt badań statutowych | NIE |
Materiał konferencyjny: | TAK |
Nazwa konferencji: | 23rd IEEE International Conference on Data Mining Workshops |
Skrócona nazwa konferencji: | 23rd ICDMW 2023 |
URL serii konferencji: | LINK |
Termin konferencji: | 1 grudnia 2023 do 4 grudnia 2023 |
Miasto konferencji: | Shanghai |
Państwo konferencji: | CHINY |
Publikacja OA: | NIE |
Abstrakty: | angielski |
In the last few years, significant progress has been made on the application of artificial intelligence (AI) within a variety of domains, especially when used as autonomous decision makers. One common concern nowadays is to quantify the trust of AI decisions. Hence, interpretability and explainability of machine learning (ML) models are a focus of current research. In our study, we developed a methodology for wheat grain classification. This, we consider to be a very important topic brought about by the necessity of cultivating cereals of high utility value. We address this issue by proposing an explainable ensemble-based model using ML classifiers, namely Support Vector Machine, Logistic Regression, eXtreme Gradient Boosting, Random Forest and Multilayer Perceptron. Each classifier learns to classify the wheat grain by learning a set of shape features extracted from X-rayed images of wheat kernels. The ensemble scheme makes final predictions on the test samples using the confidence in the predictions of the base classifiers. The interpretability of the model is explained by SHAP for the ensemble model. According to the outcome of the work, the accuracy of classification is 94% in its three class setting and the approach outperforms state-of-the-art models. Our results show that the proposed framework achieves high predictive performance while being explainable. |