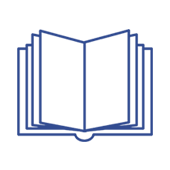
Fault detection in rotary agricultural machinery using genetic algorithm optimized multiple input – parallel – convolutional neural networks
Fragment książki (Rozdział monografii pokonferencyjnej)
MNiSW
20
Poziom I
Status: | |
Autorzy: | Sewioło Mateusz , Mystkowski Arkadiusz , Berghout Tarek, Khamari Dalila, Wolszczak Piotr, Litak Grzegorz |
Dyscypliny: | |
Aby zobaczyć szczegóły należy się zalogować. | |
Wersja dokumentu: | Drukowana | Elektroniczna |
Język: | angielski |
Strony: | 1 - 6 |
Scopus® Cytowania: | 0 |
Bazy: | Scopus | IEEE Xplore |
Efekt badań statutowych | NIE |
Finansowanie: | This research is supported by the National Centre for Research and Development (Poland), project “AGROTECH”, no: POIR.01.01.01-00-2167/20-00, title: “Research and development of agricultural machines for a sustainable harvesting of green feed with the use of smart technologies of SaMASZ company which support the concept of Agricultural Valley 4.0”, realized in 01.01.2021 – 31.12.2023, and by Bialystok University of Technology project no WZ/WE- IA/4/2023 and WI/WE-IA/14/2023 financed from a subsidy provided by the Ministry of Science and Higher Education.. |
Materiał konferencyjny: | TAK |
Nazwa konferencji: | 2023 International Conference on Engineering and Advanced Technology |
Skrócona nazwa konferencji: | ICEEAT 2023 |
URL serii konferencji: | LINK |
Termin konferencji: | 5 listopada 2023 do 7 listopada 2023 |
Miasto konferencji: | Batna |
Państwo konferencji: | ALGERIA |
Publikacja OA: | NIE |
Abstrakty: | angielski |
In this article we propose a novel method to detect faults in agricultural rotary hay tedder, while it is working in the field, using parallel–convolutional neural networks (CNNs), designed and optimized by genetic algorithm. In this case, the problem to solve is achieving as best as possible accuracy using the genetic algorithm to utilize the optimal CNNs architecture represented by each specimen. In total, the combination of the 5 vibration signals were used as the multiple CNNs inputs. Experimental results show that our method was able to reach 80% of prediction accuracy, when classifying 7 different machine states. |