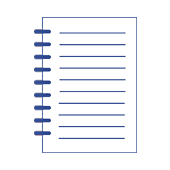
The Use of Artificial Intelligence for Quality Assessment of Refill Friction Stir Spot Welded Thin Joints
Artykuł w czasopiśmie
MNiSW
100
Lista 2024
Status: | |
Autorzy: | Kubit Andrzej, Kłosowski Grzegorz, Berezowski Wojciech |
Dyscypliny: | |
Aby zobaczyć szczegóły należy się zalogować. | |
Rok wydania: | 2024 |
Wersja dokumentu: | Drukowana | Elektroniczna |
Język: | angielski |
Numer czasopisma: | 3 |
Wolumen/Tom: | 18 |
Strony: | 45 - 57 |
Impact Factor: | 1,0 |
Web of Science® Times Cited: | 0 |
Scopus® Cytowania: | 0 |
Bazy: | Web of Science | Scopus | BazTech |
Efekt badań statutowych | NIE |
Finansowanie: | The research leading to these results has received funding from the commissioned task entitled “VIA CARPATIA Universities of Technology Network named after the President of the Republic of Poland Lech Kaczyński” contract no. MEiN/2022/DPI/2575 concluded on October 20, 2023 action entitled “In the neighborhood - interuniversity research internships and study visits.” |
Materiał konferencyjny: | NIE |
Publikacja OA: | TAK |
Licencja: | |
Sposób udostępnienia: | Otwarte czasopismo |
Wersja tekstu: | Ostateczna wersja opublikowana |
Czas opublikowania: | W momencie opublikowania |
Data opublikowania w OA: | 20 kwietnia 2024 |
Abstrakty: | angielski |
This paper presents a machine learning and image segmentation based advanced quality assessment technique for thin Refill Friction Stir Spot Welded (RFSSW) joints. In particular, the research focuses on developing a predictive support vector machines (SVM) model. The purpose of this model is to facilitate the selection of RFSSW process parameters in order to increase the shear load capacity of joints. In addition, an improved weld quality assessment algorithm based on optical analysis was developed. The research methodology includes specimen preparation stages, mechanical tests, and algorithmic analysis, culminating in a machine learning model trained on experimental data. The results demonstrate the effectiveness of the model in selecting welding process parameters and assessing weld quality, offering significant improvements compared to standard techniques. This research not only proposes a novel approach to optimizing welding parameters but also facilitates automatic quality assessment, potentially revolutionizing and spreading the application of the RFSSW technique in various industries. |