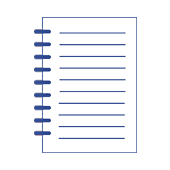
Evaluation and Comparison of Selected Machine Learning Methods for Improving Maintenance Processes
Artykuł w czasopiśmie
MNiSW
20
Lista 2024
Status: | |
Autorzy: | Antosz Katarzyna, Kulisz Monika, Husar Jozef |
Dyscypliny: | |
Aby zobaczyć szczegóły należy się zalogować. | |
Rok wydania: | 2024 |
Wersja dokumentu: | Drukowana | Elektroniczna |
Język: | angielski |
Numer czasopisma: | 8 |
Wolumen/Tom: | 58 |
Strony: | 85 - 90 |
Scopus® Cytowania: | 0 |
Bazy: | Scopus |
Efekt badań statutowych | NIE |
Materiał konferencyjny: | NIE |
Publikacja OA: | TAK |
Licencja: | |
Sposób udostępnienia: | Witryna wydawcy |
Wersja tekstu: | Ostateczna wersja opublikowana |
Czas opublikowania: | W momencie opublikowania |
Data opublikowania w OA: | 6 września 2024 |
Abstrakty: | angielski |
It is a constant objective for manufacturing companies to improve the efficiency and effectiveness of their maintenance processes. Avoiding unexpected breakdowns that result in high costs and production losses is a major concern. Not only the selection of appropriate maintenance strategies, but also the use of appropriate methods and tools to support decision making in this area is essential to achieve this goal. This article presents the possibility of using machine learning methods to develop predictive models to support decision making in maintenance management. For this purpose, three different machine learning methods have been considered: Neural Networks (NN), the k-Nearest Neighbours algorithm (KNN) and Support Vector Machines (SVM). These models were constructed using variables identified through statistical analysis as having a significant impact on the effectiveness of maintenance processes, specifically the availability of machinery in the production process. The developed models were subjected to a qualitative evaluation, which led to the identification of the SVM model as the most suitable to support decision making in the planning and execution of maintenance processes. |