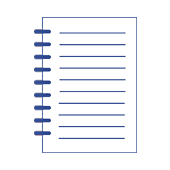
Management Decision Making in a Retail Establishment Using Machine Learning Methods
Artykuł w czasopiśmie
MNiSW
100
Lista 2024
Status: | |
Autorzy: | Bojanowska Agnieszka, Kulisz Monika, Infante-Moro Alfonso |
Dyscypliny: | |
Aby zobaczyć szczegóły należy się zalogować. | |
Rok wydania: | 2024 |
Wersja dokumentu: | Elektroniczna |
Język: | angielski |
Numer czasopisma: | 7 |
Wolumen/Tom: | 18 |
Strony: | 460 - 474 |
Impact Factor: | 1,0 |
Scopus® Cytowania: | 0 |
Bazy: | Scopus | BazTech | Google Scholar |
Efekt badań statutowych | NIE |
Materiał konferencyjny: | NIE |
Publikacja OA: | TAK |
Licencja: | |
Sposób udostępnienia: | Otwarte czasopismo |
Wersja tekstu: | Ostateczna wersja opublikowana |
Czas opublikowania: | W momencie opublikowania |
Data opublikowania w OA: | 11 września 2024 |
Abstrakty: | angielski |
Management decisions about store atmosphere, such as temperature, or light intensity in retail establishments can be made based on solutions from machine learning methods. These conditions determine whether the customer will stay in the store longer and whether his shopping cart will reach the desired high value. Previous literature research associates certain atmospheric factors with customers' propensity to make purchasing decisions and allows us to identify what influences the customer during shopping and to what extent. The article aims to reveal the feasibility of using machine learning methods to make management decisions based on store atmosphere parameters. When deciding on the conditions in a retail establishment, applicable health and safety regulations should also be considered. This was used to set limits on the input parameters for the model. The authors identified 3 atmospheric factors and, based on them, proposed two types of models: regression and classification models, predicting how long customers stay in an establishment and can classify it into categories: short, medium and long. These models can then be used to create a model that optimizes the parameters in the facility to achieve a minimum given time a customer stays in the facility. |