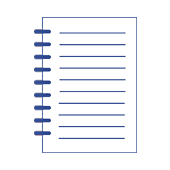
Modelling the properties of aerated concrete on the basis of raw materials and ash-and-slag wastes using machine learning paradigm
Artykuł w czasopiśmie
MNiSW
70
Lista 2024
Status: | |
Autorzy: | Rudenko Olga, Galkina Darya, Sadenova Marzhan, Beisekenov Nail, Kulisz Monika, Begentayev Meiram |
Dyscypliny: | |
Aby zobaczyć szczegóły należy się zalogować. | |
Rok wydania: | 2024 |
Wersja dokumentu: | Elektroniczna |
Język: | angielski |
Wolumen/Tom: | 11 |
Strony: | 1 - 21 |
Impact Factor: | 2,6 |
Web of Science® Times Cited: | 0 |
Bazy: | Web of Science | Google Scholar | DOAJ | CrossRef | Chemical Abstracts Service (CAS) | CLOCKSS |
Efekt badań statutowych | NIE |
Finansowanie: | The author(s) declare that financial support was received for the research, authorship, and/or publication of this article. This research is funded by the Committee of Science of the Ministry of Science and Higher Education of the Republic of Kazakhstan (Grant No. BR21882292 – “Integrated development of sustainable construction industries: innovative technologies, optimization of production, effective use of resources and creation of technological park”). |
Materiał konferencyjny: | NIE |
Publikacja OA: | TAK |
Licencja: | |
Sposób udostępnienia: | Witryna wydawcy |
Wersja tekstu: | Ostateczna wersja opublikowana |
Czas opublikowania: | W momencie opublikowania |
Data opublikowania w OA: | 22 października 2024 |
Abstrakty: | angielski |
The thermal power industry, as a major consumer of hard coal, ignificantly contributes to harmful emissions, affecting both air quality and soil health during the operation and transportation of ash and slag waste. This study presents the modeling of aerated concrete using local raw materials and ash-and-slag waste in seismic areas through machine learning techniques.A comprehensive literature review and comparative analysis of normative documentation underscore the relevance and feasibility of employing nonautoclaved aerated concrete blocks in such regions. Machine learning methods are particularly effective for disjointed datasets, with neural networks demonstrating superior performance in modeling complex relationships for predicting concrete strength and density. The results reveal that neural networks, especially those with Bayesian Regularisation, consistently outperformed decision trees, achieving higher regression values (Rstrength = 0.9587 and Rdensity = 0.91997) and lower error metrics (MSE, RMSE, RIE, MAE). This indicates their advanced capability to capture intricate non-linear patterns. The study concludes that artificial neural networks are a robust tool for predicting concrete properties, crucial for producing non-autoclaved curing wall blocks suitable for earthquake-resistant construction. Future research should focus on optimizing the balance between density and strength of blocks by enhancing the properties of aerated concrete and utilizing reliable models. |