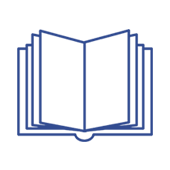
Fuzzy Ensemble Learning and Lightweight CNN for Stress Classification
Fragment książki (Materiały konferencyjne)
MNiSW
140
konferencja
Status: | |
Autorzy: | Baran Katarzyna |
Dyscypliny: | |
Aby zobaczyć szczegóły należy się zalogować. | |
Wersja dokumentu: | Elektroniczna |
Język: | angielski |
Strony: | 4741 - 4747 |
Efekt badań statutowych | NIE |
Materiał konferencyjny: | TAK |
Nazwa konferencji: | 27th European Conference on Artificial Intelligence ; 13th Conference on Prestigious Applications of Intelligent Systems |
Skrócona nazwa konferencji: | 27th ECAI 2024 ; 13th PAIS 2024 |
URL serii konferencji: | LINK |
Termin konferencji: | 19 października 2024 do 24 października 2024 |
Miasto konferencji: | Santiago de Compostela |
Państwo konferencji: | HISZPANIA |
Publikacja OA: | TAK |
Licencja: | |
Sposób udostępnienia: | Witryna wydawcy |
Wersja tekstu: | Ostateczna wersja opublikowana |
Czas opublikowania: | W momencie opublikowania |
Data opublikowania w OA: | 19 października 2024 |
Abstrakty: | angielski |
Everyday life problems, armed conflicts, pandemics, and catastrophes – these are situations that are always accompanied by stress. Its chronic form can lead to so-called stress-related illnesses. Despite the development of health prevention, many people still get sick due to stress. Therefore, it is important to seek detective and classificatory solutions for stress, which may influence its reduction or control in the future. The example of this can be the thermographic stress registration presented in this article, combined with classifica- tion using lightweight CNN and Choquet fuzzy ensemble learning. The article proposed new ensemble frameworks for stress classifica- tion based on Choquet fuzzy integral, serving as an aggregation func- tion. In the study, three pre-trained lightweight CNN models were used: MobileNetV2, Xception, and EfficientNet. The proposed fuzzy ensemble model achieves a classification accuracy above 90%. This work is of a prospective nature, with the possibility of implementing solutions in biomedical-psychological activities. |