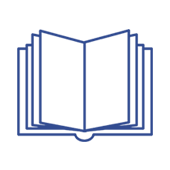
ShuffleNet and XGBoost classifier for stress reactions detection
Fragment książki (Materiały konferencyjne)
MNiSW
70
konferencja
Status: | |
Autorzy: | Baran Katarzyna |
Dyscypliny: | |
Aby zobaczyć szczegóły należy się zalogować. | |
Wersja dokumentu: | Drukowana | Elektroniczna |
Język: | angielski |
Strony: | 3771 - 3780 |
Efekt badań statutowych | NIE |
Materiał konferencyjny: | TAK |
Nazwa konferencji: | 28th International Conference on Knowledge Based and Intelligent information and Engineering Systems |
Skrócona nazwa konferencji: | 28th KES 2024 |
URL serii konferencji: | LINK |
Termin konferencji: | 11 września 2024 do 13 września 2024 |
Miasto konferencji: | Seville |
Państwo konferencji: | HISZPANIA |
Publikacja OA: | TAK |
Licencja: | |
Sposób udostępnienia: | Witryna wydawcy |
Wersja tekstu: | Ostateczna wersja opublikowana |
Czas opublikowania: | W momencie opublikowania |
Data opublikowania w OA: | 28 listopada 2024 |
Abstrakty: | angielski |
A huge problem of the society of the present century is stress. It is not possible to eliminate it completely, but it can be controlled and actions can be taken to eliminate it or build a way of coping with stress. Stress can manifest itself in a physiological as well as psychological form. It can be visible through facial expressions, which is related to emotions. Emotions, like stress, can be positive or negative. Therefore, the observation of a person’s emotions can lead to the determination of their stress response and even the intensity of stress. Emotions allow to regulate stress sensations – increase their intensity or, on the contrary, get rid of or relieve stress. Observing the rapid development of machine learning, it is worth using the resources of neural networks for stress control and monitoring purposes. Many detection techniques use complex neural networks, which at the same time require large computer resources, are time-consuming and expensive. Therefore, it is important to use and develop lightweight neural networks that do not require huge computer resources. In addition, it is worth considering the use of expensive measuring devices, while there are many handheld devices with convergent parameters on the technical market. An example is a smartphone thermal imaging camera that can provide recording accuracy, and the acquired thermal images can be a source of data. With the whole thing in mind, in this paper, the author proposes a lightweight convolutional network drawing on ShuffleNet to detect stress from thermal images of faces expressing various emotional forms. The proposed network includes additional layers and the XGBoost classifier. As a result of the calculations, the accuracy in the multi-class classification reached 87%. The research confirmed the validity and effectiveness of the proposed network and the capabilities of the detection technique. Certainly, the subject of stress and light convolutional neural networks is developmental and will be developed by the author in the future. |