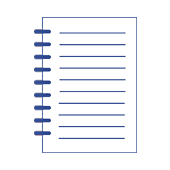
Polish dance music classification based on mel spectrogram decomposition
Artykuł w czasopiśmie
MNiSW
100
Lista 2024
Status: | |
Autorzy: | Chwaleba Kinga, Wach Weronika |
Dyscypliny: | |
Aby zobaczyć szczegóły należy się zalogować. | |
Rok wydania: | 2025 |
Wersja dokumentu: | Elektroniczna |
Język: | angielski |
Numer czasopisma: | 2 |
Wolumen/Tom: | 19 |
Strony: | 95 - 113 |
Efekt badań statutowych | NIE |
Materiał konferencyjny: | NIE |
Publikacja OA: | TAK |
Licencja: | |
Sposób udostępnienia: | Otwarte czasopismo |
Wersja tekstu: | Ostateczna wersja opublikowana |
Czas opublikowania: | W momencie opublikowania |
Data opublikowania w OA: | 1 stycznia 2025 |
Abstrakty: | angielski |
Folk dances and music are essential aspects of intangible cultural heritage identifying the history and traditions of nations. Due to dynamic changes in the social structure, many national aspects are not cultivated and therefore forgotten. There is a need to develop methods to preserve these valuable aspects of culture. There are five Polish national dances: the Polonez, the Oberek, the Mazur, the Krakowiak, and the Kujawiak that reflect key elements of Polish intangible cultural heritage. They can be observed both in the way of performing dances as well as in music. There are many preserved audio and video files that differ depending on the multiple features such as composers or versions. The primary objective of this study was to apply machine learning approaches in order to distinguish the above-mentioned music of Polish traditional dances. The audio recordings dataset consisting of 137 dances in mp3 format was created. Each recording was divided into ten-second files reflecting the characteristic elements of each dance. The transformation of sound to the Mel scale improves human auditory perception. Thus, from ev- ery recording the Mel-spectrograms were generated. For the purpose of this study the most applied classification tools were compared such as VGG16, ResNet50, DenseNet121, and MobileNetV2. To compare the performance of the selected models, the following measures were applied: accuracy, precision, recall, and F1 score. ResNet50 achieved the best testing accuracy (over 90%), while DenseNet121 had the best testing loss (0.38). |