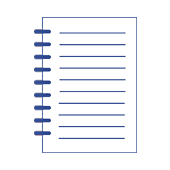
A disassembly-free method for evaluation of spiral bevel gear assembly
Artykuł w czasopiśmie
MNiSW
45
Lista A
Status: | |
Autorzy: | Jedliński Łukasz, Jonak Józef |
Dyscypliny: | |
Aby zobaczyć szczegóły należy się zalogować. | |
Rok wydania: | 2017 |
Wersja dokumentu: | Drukowana | Elektroniczna |
Język: | angielski |
Wolumen/Tom: | 88 |
Strony: | 399 - 412 |
Impact Factor: | 4,37 |
Web of Science® Times Cited: | 26 |
Scopus® Cytowania: | 30 |
Bazy: | Web of Science | Scopus | Web of Science Core Collection | Science Direct |
Efekt badań statutowych | NIE |
Materiał konferencyjny: | NIE |
Publikacja OA: | NIE |
Abstrakty: | angielski |
The paper presents a novel method for evaluation of assembly of spiral bevel gears. The examination of the approaches to the problem of gear control diagnostics without disassembly has revealed that residual processes in the form of vibrations (or noise) are currently the most suitable to this end. According to the literature, contact pattern is a complex parameter for describing gear position. Therefore, the task is to determine the correlation between contact pattern and gear vibrations. Although the vibration signal contains a great deal of information, it also has a complex spectral structure and contains interferences. For this reason, the proposed method has three variants which determine the effect of preliminary processing of the signal on the results. In Variant 2, stage 1, the vibration signal is subjected to multichannel denoising using a wavelet transform (WT), and in Variant 3 – to a combination of WT and principal component analysis (PCA). This denoising procedure does not occur in Variant 1. Next, we determine the features of the vibration signal in order to focus on information which is crucial regarding the objective of the study. Given the lack of unequivocal premises enabling selection of optimum features, we calculate twenty features, rank them and finally select the appropriate ones using an algorithm. Diagnostic rules were created using artificial neural networks. We investigated the suitability of three network types: multilayer perceptron (MLP), radial basis function (RBF) and support vector machine (SVM). |