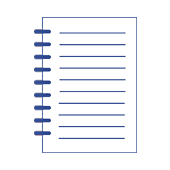
Classification of user performance in the Ruff Figural Fluency Test based on eye-tracking features
Materiały konferencyjne
MNiSW
15
WOS
Status: | |
Autorzy: | Borys Magdalena, Barakate Sara, Hachmoud Karim, Plechawska-Wójcik Małgorzata, Krukow Paweł, Kamiński Marek |
Dyscypliny: | |
Aby zobaczyć szczegóły należy się zalogować. | |
Rok wydania: | 2017 |
Wersja dokumentu: | Drukowana | Elektroniczna |
Język: | angielski |
Wolumen/Tom: | 15 |
Numer artykułu: | 2002 |
Strony: | 1 - 7 |
Web of Science® Times Cited: | 3 |
Bazy: | Web of Science | Google Scholar | EBSCO |
Efekt badań statutowych | NIE |
Materiał konferencyjny: | TAK |
Nazwa konferencji: | 2nd International Conference of Computational Methods in Engineering Science |
Skrócona nazwa konferencji: | CMES’17 |
URL serii konferencji: | LINK |
Termin konferencji: | 23 listopada 2017 do 25 listopada 2017 |
Miasto konferencji: | Lublin |
Państwo konferencji: | POLSKA |
Publikacja OA: | TAK |
Licencja: | |
Sposób udostępnienia: | Otwarte czasopismo |
Wersja tekstu: | Ostateczna wersja opublikowana |
Czas opublikowania: | W momencie opublikowania |
Data opublikowania w OA: | 15 grudnia 2017 |
Abstrakty: | angielski |
Cognitive assessment in neurological diseases represents a relevant topic due to its diagnostic significance in detecting disease, but also in assessing progress of the treatment. Computer-based tests provide objective and accurate cognitive skills and capacity measures. The Ruff Figural Fluency Test (RFFT) provides information about non-verbal capacity for initiation, planning, and divergent reasoning. The traditional paper form of the test was transformed into a computer application and examined. The RFFT was applied in an experiment performed among 70 male students to assess their cognitive performance in the laboratory environment. Each student was examined in three sequential series. Besides the students’ performances measured by using in app keylogging, the eye-tracking data obtained by non-invasive video-based oculography were gathered, from which several features were extracted. Eye-tracking features combined with performance measures (a total number of designs and/or error ratio) were applied in machine learning classification. Various classification algorithms were applied, and their accuracy, specificity, sensitivity and performance were compared. |