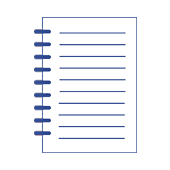
Application of selected Levy processes for degradation modelling of long range mine belt using real-time data
Artykuł w czasopiśmie
MNiSW
30
Lista A
Status: | |
Autorzy: | Vališ David, Mazurkiewicz Dariusz |
Dyscypliny: | |
Aby zobaczyć szczegóły należy się zalogować. | |
Rok wydania: | 2018 |
Wersja dokumentu: | Drukowana | Elektroniczna |
Arkusze wydawnicze: | 0,64 |
Język: | angielski |
Numer czasopisma: | 4 |
Wolumen/Tom: | 18 |
Strony: | 1430 - 1440 |
Impact Factor: | 2,846 |
Web of Science® Times Cited: | 60 |
Scopus® Cytowania: | 72 |
Bazy: | Web of Science | Scopus | BazTEch | EBSCO |
Efekt badań statutowych | NIE |
Materiał konferencyjny: | NIE |
Publikacja OA: | NIE |
Abstrakty: | angielski |
When analysing big data generated by a typical diagnostic system, the maintenance operator has to deal with several problems, including a substantial number of data appearing every second. Maintenance systems, especially those in mining industry additionally require the operator to make reliable predictions and decisions under uncertainty. All this create so called information overload problem, which can be solved in data mining with the use of existing data reduction techniques. Unfortunately, with complex mining machinery operating under diverse conditions more advanced approaches are needed. Effective solutions can be found among non-trivial degradation assessment techniques provided which shall be properly applied. This work proposes new methods to modelling specific system degradation and prognosis for system failure occurrence. The approach presented here does not rely on typical statistical assumptions. This paper relates to mathematical modelling of real diagnostic data with the use of selected stochastic processes – types of Wiener process and Ornstein–Uhlenbeck process. The main novelty and contribution is in the specific forms of above mentioned processes, in the ways how the process parameters were estimated and also in realistic correlation of proposed models to the studied system. Simulated and real case results show that the proposed robust functional analysis reduces bias and provides more accurate false fault detection rates, as compared to the previous method. We hope the outcomes provide applicable inputs for more effective principles of system operation, predictive maintenance policy and risk assessment. |