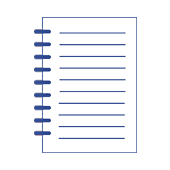
Properties of the Surface Layer After Trochoidal Milling and Brushing: Experimental Study and Artificial Neural Network Simulation
Artykuł w czasopiśmie
MNiSW
100
Lista 2021
Status: | |
Autorzy: | Kulisz Monika, Zagórski Ireneusz, Matuszak Jakub, Kłonica Mariusz |
Dyscypliny: | |
Aby zobaczyć szczegóły należy się zalogować. | |
Rok wydania: | 2020 |
Wersja dokumentu: | Drukowana | Elektroniczna |
Język: | angielski |
Numer czasopisma: | 1 |
Wolumen/Tom: | 10 |
Numer artykułu: | 75 |
Strony: | 1 - 26 |
Impact Factor: | 2,679 |
Web of Science® Times Cited: | 19 |
Scopus® Cytowania: | 22 |
Bazy: | Web of Science | Scopus |
Efekt badań statutowych | NIE |
Finansowanie: | The project/research was financed in the framework of the project Lublin University of Technology-Regional Excellence Initiative, funded by the Polish Ministry of Science and Higher Education (contract no. 030/RID/2018/19). |
Materiał konferencyjny: | NIE |
Publikacja OA: | TAK |
Licencja: | |
Sposób udostępnienia: | Witryna wydawcy |
Wersja tekstu: | Ostateczna wersja opublikowana |
Czas opublikowania: | W momencie opublikowania |
Data opublikowania w OA: | 20 grudnia 2019 |
Abstrakty: | angielski |
e aim of this study was to investigate the effect of milling and brushing cutting data settings on the surface geometry and energy parameters of two Mg alloy substrates: AZ91D and AZ31. In milling, the cutting speed and the trochoidal step were modified (vc = 400–1200 m/min and str = 5–30%) to investigate how they affect selected 2D (Rz, Rku, Rsk, RSm, Ra) and 3D (Sa, Sz, Sku, Ssk) roughness parameters. The brushing treatment was carried out at constant parameters: n = 5000 rev/min, vf = 300 mm/min, ap = 0.5 mm. The surface roughness of specimens was assessed with the Ra, Rz, and RSm parameters. The effects of the two treatments on the workpiece surface were analyzed comparatively. It was found that the roughness properties of the machined surface may be improved by the application of a carbide milling cutter and ceramic brush. The use of different machining data was also shown to impact the surface free energy and its polar component of Mg alloy specimens. Complementary to the results from the experimental part of the study, the investigated machining processes were modelled by means of statistical artificial neural networks (the radial basis function and multi-layered perceptron). The artificial neural networks (ANNs) were shown to perform well as a tool for the prediction of Mg alloy surface roughness parameters and the maximum height of the profile (Rz) after milling and brushing. |