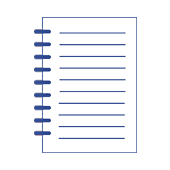
The Use of Time-Frequency Moments as Inputs of LSTM Network for ECG Signal Classification
Artykuł w czasopiśmie
MNiSW
100
Lista 2021
Status: | |
Autorzy: | Kłosowski Grzegorz, Rymarczyk Tomasz, Wójcik Dariusz, Skowron Stanisław, Cieplak Tomasz, Adamkiewicz Przemysław |
Dyscypliny: | |
Aby zobaczyć szczegóły należy się zalogować. | |
Rok wydania: | 2020 |
Wersja dokumentu: | Drukowana | Elektroniczna |
Język: | angielski |
Numer czasopisma: | 9 |
Wolumen/Tom: | 9 |
Numer artykułu: | 1452 |
Strony: | 1 - 22 |
Impact Factor: | 2,397 |
Web of Science® Times Cited: | 49 |
Scopus® Cytowania: | 57 |
Bazy: | Web of Science | Scopus |
Efekt badań statutowych | NIE |
Materiał konferencyjny: | NIE |
Publikacja OA: | TAK |
Licencja: | |
Sposób udostępnienia: | Witryna wydawcy |
Wersja tekstu: | Ostateczna wersja opublikowana |
Czas opublikowania: | W momencie opublikowania |
Data opublikowania w OA: | 6 września 2020 |
Abstrakty: | angielski |
This paper refers to the method of using the deep neural long-short-term memory (LSTM)network for the problem of electrocardiogram (ECG) signal classification. ECG signals contain a lot ofsubtle information analyzed by doctors to determine the type of heart dysfunction. Due to the largenumber of signal features that are difficult to identify, raw ECG data is usually not suitable for use inmachine learning. The article presents how to transform individual ECG time series into spectralimages for which two characteristics are determined, which are instantaneous frequency and spectralentropy. Feature extraction consists of converting the ECG signal into a series of spectral images usingshort-term Fourier transformation. Then the images were converted using Fourier transform again totwo signals, which includes instantaneous frequency and spectral entropy. The data set transformedin this way was used to train the LSTM network. During the experiments, the LSTM networks weretrained for both raw and spectrally transformed data. Then, the LSTM networks trained in this waywere compared with each other. The obtained results prove that the transformation of input signalsinto images can be an effective method of improving the quality of classifiers based on deep learning. |