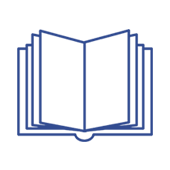
Detection of Road Artefacts Using Fuzzy Adaptive Thresholding
Fragment książki (Materiały konferencyjne)
MNiSW
140
konferencja
Status: | |
Autorzy: | Badurowicz Marcin, Montusiewicz Jerzy, Karczmarek Paweł |
Dyscypliny: | |
Aby zobaczyć szczegóły należy się zalogować. | |
Wersja dokumentu: | Drukowana | Elektroniczna |
Język: | angielski |
Strony: | 1 - 8 |
Web of Science® Times Cited: | 1 |
Scopus® Cytowania: | 7 |
Bazy: | Web of Science | Scopus | IEEE Xplore |
Efekt badań statutowych | NIE |
Finansowanie: | Funded by the National Science Centre, Poland under CHIST-ERA programme (Grant no. 2018/28/Z/ST6/00563). |
Materiał konferencyjny: | TAK |
Nazwa konferencji: | IEEE International Conference onFuzzy Systems (FUZZ-IEEE) 2020 |
Skrócona nazwa konferencji: | FUZZ-IEEE 2020 |
URL serii konferencji: | LINK |
Termin konferencji: | 19 lipca 2020 do 24 lipca 2020 |
Miasto konferencji: | Glasgow |
Państwo konferencji: | WIELKA BRYTANIA |
Publikacja OA: | NIE |
Abstrakty: | angielski |
In this paper the authors are proposing approximate method for road artefacts detection and their location by analyzing acceleration values recorded in the car during driving over the road fragment using the smartphone mounted in the car. The new method called F-THRESH has been introduced, which is adaptively adjusting threshold for road artefacts detection by the fuzzy system means, allowing for outlier detection in chaotic time streams. First, the road quality is being calculated, then the difference between the current data point and mean acceleration is calculated and those two values are used as the input for the fuzzy system, which is calculating threshold to classify data point as an outlier. The proposed method has been compared to the previously implemented method and has an accuracy over 94% with 1.3% of False Positive Rate for the same problem which makes it a great candidate to be implemented in the IoT Edge scenarios, for reducing amount of data being sent to the cloud analyzing system. |