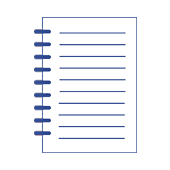
Historical Buildings Dampness Analysis Using Electrical Tomography and Machine Learning Algorithms
Artykuł w czasopiśmie
MNiSW
140
Lista 2021
Status: | |
Autorzy: | Rymarczyk Tomasz, Kłosowski Grzegorz, Hoła Anna, Hoła Jerzy, Sikora Jan, Tchórzewski Paweł, Skowron Łukasz |
Dyscypliny: | |
Aby zobaczyć szczegóły należy się zalogować. | |
Rok wydania: | 2021 |
Wersja dokumentu: | Drukowana | Elektroniczna |
Język: | angielski |
Numer czasopisma: | 5 |
Wolumen/Tom: | 14 |
Strony: | 1 - 25 |
Impact Factor: | 3,252 |
Web of Science® Times Cited: | 29 |
Scopus® Cytowania: | 33 |
Bazy: | Web of Science | Scopus |
Efekt badań statutowych | NIE |
Finansowanie: | This research was funded by The National Centre for Research and Development, grant number POIR.01.01.01-00-0167/15. |
Materiał konferencyjny: | NIE |
Publikacja OA: | TAK |
Licencja: | |
Sposób udostępnienia: | Witryna wydawcy |
Wersja tekstu: | Ostateczna wersja opublikowana |
Czas opublikowania: | W momencie opublikowania |
Data opublikowania w OA: | 27 stycznia 2021 |
Abstrakty: | angielski |
The article deals with the problem of detecting moisture in the walls of historical build-ings. As part of the presented research, the following four methods based on mathematical model-ing and machine learning were compared: total variation, least-angle regression, elastic net, and artificial neural networks. Based on the simulation data, the systems for the reconstruction of “pixel by pixel” tomographic images were trained. In order to test the reconstructive algorithms obtained during the research, images were generated based on real measurements and simulation cases. The method comparison was performed on the basis of three indicators: mean square error, relative im-age error, and image correlation coefficient. The above indicators were applied to four selected var-iants that corresponded to various parts of the walls. The variants differed in the dimensions of the tested wall sections, the number of electrodes used, and the resolution of the 3D image meshes. In all analyzed variants, the best results were obtained using the elastic net algorithm. In addition, all machine learning methods generated better tomographic reconstructions than the classic Total Var-iation method. |