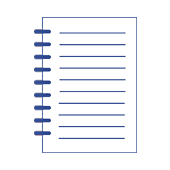
Comparison of Machine Learning Methods in Electrical Tomography for Detecting Moisture in Building Walls
Artykuł w czasopiśmie
MNiSW
140
Lista 2021
Status: | |
Autorzy: | Rymarczyk Tomasz, Kłosowski Grzegorz, Hoła Anna, Sikora Jan, Wołowiec Tomasz, Tchórzewski Paweł, Skowron Stanisław |
Dyscypliny: | |
Aby zobaczyć szczegóły należy się zalogować. | |
Rok wydania: | 2021 |
Wersja dokumentu: | Drukowana | Elektroniczna |
Język: | angielski |
Numer czasopisma: | 10 |
Wolumen/Tom: | 14 |
Numer artykułu: | 2777 |
Strony: | 1 - 22 |
Impact Factor: | 3,252 |
Web of Science® Times Cited: | 34 |
Scopus® Cytowania: | 40 |
Bazy: | Web of Science | Scopus | Ei Compendex | RePEc | AGRIS | Inspec | CAPlus / SciFinder | and many other databases |
Efekt badań statutowych | NIE |
Materiał konferencyjny: | NIE |
Publikacja OA: | TAK |
Licencja: | |
Sposób udostępnienia: | Witryna wydawcy |
Wersja tekstu: | Ostateczna wersja opublikowana |
Czas opublikowania: | W momencie opublikowania |
Data opublikowania w OA: | 12 maja 2021 |
Abstrakty: | angielski |
This paper presents the results of research on the use of machine learning algorithms and electrical tomography in detecting humidity inside the walls of old buildings and structures. The object of research was a historical building in Wrocław, Poland, built in the first decade of the 19th century. Using the prototype of an electric tomograph of our own design, a number of voltage measurements were made on selected parts of the building. Many algorithmic methods have been preliminarily analyzed. Ultimately, the three models based on machine learning were selected: linear regression with SVM (support vector machine) learner, linear regression with least squares learner, and a multilayer perceptron neural network. The classical Gauss–Newton model was also used in the comparison. Both the experiments based on real measurements and simulation data showed a higher efficiency of machine learning methods than the Gauss–Newton method. The tomographic methods surpassed the point methods in measuring the dampness in the walls because they show a spatial image of the interior and not separate points of the examined cross-section. Research has shown that the selection of a machine learning model has a large impact on the quality of the results. Machine learning has a greater potential to create correct tomographic reconstructions than traditional mathematical methods. In this research, linear regression models performed slightly worse than neural networks. |