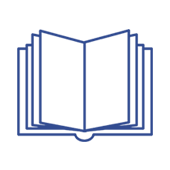
Analysis of Sub-Integral Functions in the Aggregation of Classification Results Using Generalizations of the Choquet Integral on the Example of Emotion Classification
Fragment książki (Materiały konferencyjne)
MNiSW
140
konferencja
Status: | |
Autorzy: | Karczmarek Paweł, Powroźnik Paweł, Skublewska-Paszkowska Maria, Przyłucki Sławomir, Łukasik Edyta |
Dyscypliny: | |
Aby zobaczyć szczegóły należy się zalogować. | |
Wersja dokumentu: | Drukowana | Elektroniczna |
Język: | angielski |
Strony: | 1 - 8 |
Web of Science® Times Cited: | 0 |
Scopus® Cytowania: | 2 |
Bazy: | Web of Science | Scopus |
Efekt badań statutowych | NIE |
Materiał konferencyjny: | TAK |
Nazwa konferencji: | IEEE World Congress on Computational Intelligence 2022 ; IEEE International Conference on Fuzzy Systems (FUZZ-IEEE) 2022 |
Skrócona nazwa konferencji: | IEEE WCCI 2022 ; FUZZ-IEEE 2022 |
URL serii konferencji: | LINK |
Termin konferencji: | 18 lipca 2022 do 23 lipca 2022 |
Miasto konferencji: | Padwa |
Państwo konferencji: | WŁOCHY |
Publikacja OA: | NIE |
Abstrakty: | angielski |
Speech emotion recognition is a complicated and challenging task in the human-computer interaction. Its performance depends on the relevance of the considered features in addition to the extent by which the speakers express their emotions. The well-known methods for determining the characteristics of a speech signal for the classification of emotional states are: The Mel Frequency Cepstral Coefficients, spectral methods like Short Time Fourier Transform and wavelet transform. However, the conventional approach to classifying emotions encounters problems, such as lack of sharp boundaries between separate emotional states, features correlation or expressing the same emotions differently depending on gender, culture orage. To prevent these issues, the fuzzy techniques such as an aggregation on a basis of the Choquet integral can be efficiently applied. Therefore, the main goal of this study is to determine the most efficient integrand of the popular Choquet integral generalizations. To find such a kind of functions, we run six separate tests for non-fuzzy classifiers: Multilayer Perceptron, Naive Bayes Network, Decision Trees, Probabilistic Neural Network, Random Forest and fuzzy ones: Fuzzy Multilayer Perceptron, Fuzzy Rule Classifier and Fuzzy Decision Trees. Additionally, the aggregation of the classification processes using 25 families of t-norms serving as integrands was performed. The results obtained in this extensive set of numerical experiments helped to establish the most effective operators which can be applied successfully in the multi-modal emotion recognition tasks. The used aggregation increased the emotions recognition accuracy for non-fuzzy classifiers from 11.10% to 15.50%, and for fuzzy classifiers from 3.31% to 6.00% depending on tested dataset. |