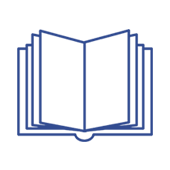
Aggregation of Tennis Groundstrokes on the Basis of the Choquet Integral and Its Generalizations
Fragment książki (Materiały konferencyjne)
MNiSW
140
konferencja
Status: | |
Autorzy: | Skublewska-Paszkowska Maria, Powroźnik Paweł, Karczmarek Paweł, Łukasik Edyta |
Dyscypliny: | |
Aby zobaczyć szczegóły należy się zalogować. | |
Wersja dokumentu: | Drukowana | Elektroniczna |
Język: | angielski |
Strony: | 1 - 8 |
Web of Science® Times Cited: | 0 |
Scopus® Cytowania: | 4 |
Bazy: | Web of Science | Scopus |
Efekt badań statutowych | NIE |
Finansowanie: | The research programme titled "Biomechanical parameters of athletes in the individual exercises" based on the analysis of 3D motion data and EMG, realised in the Laboratory of Motion Analysis and Interface Ergonomics was approved by the Commission for Research Ethics, No. 2/2016 dated 8.04.2016. The authors would like to thank the Tennis Academy POL-STAR Student Sports Club, and KS-WINNER Club for their support. |
Materiał konferencyjny: | TAK |
Nazwa konferencji: | IEEE World Congress on Computational Intelligence 2022 ; IEEE International Conference on Fuzzy Systems (FUZZ-IEEE) 2022 |
Skrócona nazwa konferencji: | IEEE WCCI 2022 ; FUZZ-IEEE 2022 |
URL serii konferencji: | LINK |
Termin konferencji: | 18 lipca 2022 do 23 lipca 2022 |
Miasto konferencji: | Padwa |
Państwo konferencji: | WŁOCHY |
Publikacja OA: | NIE |
Abstrakty: | angielski |
This paper focuses on the aggregation of two tennis groundstrokes: Forehand and backhand, which are performed in every match and training session. Recognition of tennis movements is very challenging task. Therefore it is important to correctly classify the proper patterns of various tennis players. This kind of study may be a part of training or development of a player’s skills. In this study, Support Vector Machines, Multilayer Perceptron, and Spatial-Temporal Graph Convolu- tional Neural Networks are applied to fi nd a match and put the move trajectories into the proper classes. The images obtained from three dimensional data recorded using the Vicon optical motion capture system are the input data for the classifi ers. The images containing forehand and backhand shots are divided into two phases: The preparation and the shot together with the racket swinging as the fi nishing element of the move. Due to the problems with strict classifi cation of these data, the input is also fuzzy. In order to improve the accuracy of the forehand and backhand recognition, the non-typical Choquet-like integral aggregation functions are applied as well as traditional aggregation operators like median, or voting. In particular, the so-called pre-aggregation operators with specifi c t-norms, overlap functions and modifi cation of the shape of the function under the integral sign give the best results, reaching accuracy at the level of 98.88%, which is better than the above mentioned individual classifi ers. |