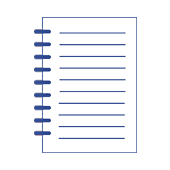
Isolation Forest Based on Minimal Spanning Tree
Artykuł w czasopiśmie
MNiSW
100
Lista 2021
Status: | |
Autorzy: | Gałka Łukasz, Karczmarek Paweł, Tokovarov Mikhail |
Dyscypliny: | |
Aby zobaczyć szczegóły należy się zalogować. | |
Rok wydania: | 2022 |
Wersja dokumentu: | Drukowana | Elektroniczna |
Język: | angielski |
Wolumen/Tom: | 10 |
Strony: | 74175 - 74186 |
Impact Factor: | 3,9 |
Web of Science® Times Cited: | 8 |
Scopus® Cytowania: | 9 |
Bazy: | Web of Science | Scopus |
Efekt badań statutowych | NIE |
Materiał konferencyjny: | NIE |
Publikacja OA: | TAK |
Licencja: | |
Sposób udostępnienia: | Witryna wydawcy |
Wersja tekstu: | Ostateczna wersja opublikowana |
Czas opublikowania: | W momencie opublikowania |
Data opublikowania w OA: | 13 lipca 2022 |
Abstrakty: | angielski |
Detecting anomalies in data sets has been one of the most studied issues in modern data analysis. Therefore, there is a plethora of applications in a very wide range of fields of science and technology. One of the most frequently used anomaly detection methods is Isolation Forest. In this study, we propose a novel efficient approach based on this technique. In order to improve the classification accuracy of the base method, we make two-fold modifications. First, we propose a change of the technique of building isolation trees to merge nodes by minimal spanning tree algorithm. The second change is based on a modification of the function assessing the anomaly of the analyzed element (data record) to sum of factors correlated with tree height and nearest point distance. In the series of comprehensive computational experiments, the proposed method has proven to produce better results than other compared state-of-the-art methods available in popular data mining programming libraries. It is worth stressing that the final version of the new method in comparison to original Isolation Forest is 2.9% better in terms of AUC measure. |