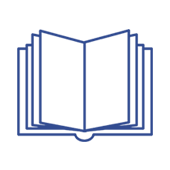
Experimental evaluation of the accuracy of an ensemble of fuzzy methods for classification of episodes in bipolar disorder
Fragment książki (Materiały konferencyjne)
MNiSW
140
konferencja
Status: | |
Autorzy: | Kaczmarek-Majer Katarzyna , Kiersztyn Adam |
Dyscypliny: | |
Aby zobaczyć szczegóły należy się zalogować. | |
Wersja dokumentu: | Drukowana | Elektroniczna |
Język: | angielski |
Strony: | 1 - 7 |
Web of Science® Times Cited: | 2 |
Scopus® Cytowania: | 2 |
Bazy: | Web of Science | Scopus |
Efekt badań statutowych | NIE |
Materiał konferencyjny: | TAK |
Nazwa konferencji: | IEEE World Congress on Computational Intelligence 2022 ; IEEE International Conference on Fuzzy Systems (FUZZ-IEEE) 2022 |
Skrócona nazwa konferencji: | IEEE WCCI 2022 ; FUZZ-IEEE 2022 |
URL serii konferencji: | LINK |
Termin konferencji: | 18 lipca 2022 do 23 lipca 2022 |
Miasto konferencji: | Padwa |
Państwo konferencji: | WŁOCHY |
Publikacja OA: | NIE |
Abstrakty: | angielski |
Clinical practice confirms that speech can support the diagnosis of several mental disorders. For example, reduced speech activity, changes in specific voice features, and pause- related measures were found to be sensitive markers of depressive symptoms. Considering the possibility of continuous speech data collection via a smartphone app, voice analysis has great potential for monitoring mental states. Nevertheless, there is still a need to select the most effective validation approaches for solving the task of predicting the mental state. Those validation approaches shall consider that the data collected from sensors and the response variables considered in this BD application problem are subject to various sources of uncertainty. The aim of the study is to perform an experimental evaluation of the accuracy of top-performing crisp and fuzzy methods, such as Naive Bayes Network, SOTA algorithm, Fuzzy Rule, Probabilistic Neural Network, Decision Tree, Gradient Boosted Tree, Random Forest, Tree Ensemble, and an ensemble approach that combines them. Various training and testing scenarios are considered for each of these methods, consisting of a given percentage of all observations. Additionally, the results from multiple methods are aggregated using the dominant function. Thus, the most frequent rating is taken and a metric based on fuzzy numbers is also considered for comparative purposes. The preliminary results of numerical experiments are promising. The sensitive point is the vicinity of the threshold of transition to a disease state. It should be noted that due to minor differences inherent in such cases, it seems intuitive to use fuzzy numbers to determine the patient’s assessment. Experiments confirmed also that the ranking of methods depends on the choice of the training set and evaluation metric. |